Update #45: Baidu's ChatGPT Rival and Machine Love
Baidu announces Ernie Bot + a history of US-China AI competition, and Joel Lehman explores a notion of love fit for machines to embody.
Welcome to the 45th update from the Gradient! If you’re new and like what you see, subscribe and follow us on Twitter :) You’ll need to view this post on Substack to see the full newsletter!
Want to write with us? Send a pitch using this form.
News Highlight: Baidu’s ChatGPT Rival, and a Brief History of the US-China Technology Race
Summary
If you see a new headline each week about US restrictions on Chinese technologies and wonder, “how did we get here?”, you’re reading the right article. As OpenAI’s ChatGPT has recently made waves around the world, Chinese tech giant Baidu is now set to release its own AI-based chatbot, named Ernie Bot.
In addition to discussing the ChatGPT rival, we’ll trace the origins of today’s US-China competition that has not only impacted AI research, but also informed national strategies and international trade. Our brief (and incomplete) history of this conflict begins in 2016 and culminates with President Biden’s budget proposal from March 2023 that calls for billions in funding specifically to outcompete China in various technological sectors and advance “American prosperity” across the globe.
Background
OpenAI’s ChatGPT has recently gained fame around the globe, introducing the world to AI-powered chatbots that can learn various linguistic skills. Aiming to compete with the technology, Baidu recently released its plans to build its own chatbot, “Ernie Bot,” which will be integrated across all of Baidu’s operations, including its smart car, smart speaker, search, and cloud services. The chatbot arms race going global is just another phase in ongoing competition between the US and China AI ecosystems. The history of this conflict intersects with international trade, and geopolitical tensions between the two countries—how did we get here?
In an interview with Wired magazine, Chinese AI pioneer Dr. Kai-Fu Lee traces this history to the spring of 2016 when DeepMind released AlphaGo, a machine learning model that could beat humans at the highly complex game of Go. While the US received AlphaGo’s victory over Lee Sedol as another milestone in the advancement of AI, the match captured the imagination of technologists and researchers in China. The event struck a nerve, as Go was invented and played primarily in Asia, but had been mastered by an algorithm made by companies in the West.
In July 2017, China unveiled its Next Generation Artificial Intelligence Development Plan, which laid out a roadmap for China to emerge as a global technological leader by 2030. With the signal from Beijing, both industry and state governments saw increased investments and support for advancing emerging technologies such as AI, and 5G communications. In October 2017, Chinese President Xi Jinping explicitly named AI as one of the core technologies to develop China in a speech to the Communist Party Congress. To quote Dr. Kai-Fu Lee’s book “AI Superpowers”, “If AlphaGo was China’s Sputnik moment, the government’s AI plan was like President John F. Kennedy’s landmark speech calling for America to land a man on the moon.”
China has since issued multiple other policy statements and laws, but it has not stopped at the written word. It is well known that apps like WeChat and other data collection sources have allowed state-backed Chinese companies to develop powerful supervised learning algorithms. In particular, China is the world’s largest facial recognition dealer, ahead of the US. And China has not slept on the impact of recent AI technologies on their population. Recent governance initiatives have targeted recommendation systems, demanding providers ensure their algorithms are explainable when harmful to users and do not harm the public interest. Three other recent governance initiatives focus on rules for online algorithms, tools for testing and certifying trustworthy AI systems, and establishing AI ethics principles.
In the United States, while federal agencies have been funding AI research for decades, funding for AI companies has steadily grown since 2011 along with investments in basic research. A study from Brookings showed that from 2017 to 2022, the US Government distributed more than $1 billion in research funding for AI. In 2019, the National Security Commission on AI was created to deliver recommendations to “responsibly use AI for national security and defense, defend against AI threats, and promote AI innovation.” As the US and China increased investment in emerging technologies, they eventually dominated different domains. A Washington Post article analyzed the US’s and China’s growth in multiple sectors over the course 5 years and found that China leads in fields such as 5G telecom tech, solar panels, and commercial drones, but the US has held its ground in semiconductors, electric vehicles, and game development. Responding to China steadily gaining ground even in the sectors it was behind, the United States began imposing restrictions on the import of Chinese tech and the export of American tech to China, specifically within the domains of semiconductors and software.
In May 2019, the Trump administration limited Chinese telecom giant Huawei from purchasing specialized US equipment without special permissions and banned their technology from being used on national security grounds; In September 2020, the White House placed restrictions on Semiconductor Manufacturing International Corporation – China’s most advanced chip manufacturer – citing an increased risk of military use of their products by the Chinese government. On Jan 5, 2021, former President Trump banned 8 Chinese apps such as Alipay and WeChat from the US due to suspected espionage. While this order was revoked by Biden, the current administration has continued Trump’s policy of tightening technological trade with China. On November 28, 2022, the US restricted the sale of high-end semiconductor chips, such as the NVIDIA A100 and H100 GPUs, which are “data-center-grade” chips typically used to build large-scale machine learning infrastructure. Additionally, in December 2022, the White House banned the sale of military and surveillance tech to China, adding 36 entities to a US export blacklist. Recent reports from February 2023 suggest that the Biden administration is considering imposing restrictions on certain US investments in Chinese tech companies.
The impact of the above restrictions on technological growth in China is still debatable. A study from the Australian Strategic Policy Institute released on March 3rd showed that China has a “stunning lead” in 37 out of 44 emerging technologies as compared to Western democracies. Consequently, the White House is showing no inclination to alter its current China strategy. In his recent budget proposal released on March 9th, President Biden presented his plan to “Invest in New Ways to Out-Compete China”, supporting nearly $6 Billion in funding to strengthen the US’s role in the Indo-Pacific. The proposal also “prioritizes China as America’s pacing challenge” and views the People’s Republic of China as the US’s “only competitor with both the intent to reshape the international order and increasingly, the economic, diplomatic, and technological power to do it”. These statements strongly underline the White House’s intent to not just advance American interests in emerging technologies but also to actively deter China from doing the same on the global stage.
The conflict between two of the biggest economies in the world has spanned almost a decade and includes more than we can write about. American big tech remains banned in China, Baidu and Pony.ai compete with Waymo and Cruise as some of the only companies in the world offering fully unsupervised autonomous driving systems, and the US Congress debates banning TikTok. I would only expect to see more regulations and restrictions in the years to come and hope that the exchange of academic knowledge and talent is not impacted in this process.
Editor Comments
Daniel: It’s always worth noting that while US-China AI competition is often at the forefront of news coverage, AI geopolitics isn’t a binary matter and I think the framing of an “AI Cold War” can be counterproductive. Prof Joanna Bryson thinks Chinese AI capacity has been exaggerated while that of other global regions has been deprecated. The quick follow-ups we saw to GPT-3 indicate multiple countries have the interest and capability to build models at large scale, though the US’s kneecap of China’s semiconductor industry will throw things off balance in one respect. I wrote at the end of 2021 about how foundation models were starting to act with the “AI nationalism” phenomenon—I expect ChatGPT and its ilk to contribute to this trend as well.
Research Highlight: Machine Love
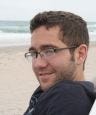
Summary
Machine learning systems are constantly improving at optimizing simple metrics like user retention, but such metrics often fail to align with improving human welfare. his A recent paper by Joel Lehman highlights the need for richer models of human flourishing in ML, provides a framework for how ML systems might support human flourishing, and demonstrates that current language models can enable qualitative humanistic principles to an extent.
Overview
Machine learning systems massively influence people’s day-to-day lives, but often come with negative effects. For instance, there is evidence that recommender systems in social media may help spread disinformation, cause certain types of mental illness, or encourage addictive behaviors. Such systems are often developed to maximize engagement — that is, time spent using the service. As noted by Lehman, the assumed model of human behavior built into these systems resembles revealed preference theory, in which a person’s external choices reveal their actual internal preferences.
Maslow’s Hierarchy of Needs
While engagement can readily be operationalized and optimized for, revealed preference theory is flawed in many senses for capturing human desires. Instead, Lehman argues that the popular, albeit imperfect, Maslow’s hierarchy of needs is a better model of human flourishing. In Maslow’s hierarchy of needs, humans pursue five different types of needs; the needs that come earlier in the hierarchy are more important (e.g. physiological needs like food come before esteem-related needs like career prestige). To support language model- (LM) based experiments that highlight the limitations of optimizing for revealed preferences, Lehman develops a synthetic environment called Maslow’s Gridworld. He shows that when high-engagement sections (i.e. those that are highly attractive but do not provide much of the need that they target) are added to the grid environment, agents spend a lot of time in the high-engagement sections, at the expense of their own flourishing (as measured by the average number of needs in Maslow’s hierarchy that are met).
Since previous approaches like optimizing for engagement are not aligned with increasing human flourishing, Lehman proposes to study a notion of machine love to guide the actions of ML systems. This is motivated by the fact that many cultures, fields of study, and spiritual traditions have identified and explored love as one of the most important parts of human life. Importantly, machine love need not, and probably should not, closely resemble love between humans. Human love is difficult to understand, and we do not need machines to simulate affect for them to act in loving ways towards humans. Instead of emotional or romantic aspects of love, Lehman focuses on “love as a practical skill”. Here, he utilizes Eric Fromm’s framework, which proposes that practical skills underlying love can be classified under care, responsibility, respect, and knowledge (summarized in table below). For instance, respect means that you should help and support the object of your love in their own personal desires.
Given this prototypical framework of love, Lehman explores how LMs like variants of GPT-3 may be able to implement loving action. He finds that LMs encode some notion of care (in the sense of Fromm): when given transcripts of an agent acting in Maslow’s Gridworld, the LM can predict whether the agent is flourishing or not (e.g. by noting whether the agent is spending too much time on social media). However, such predictions are based on general priors on social media’s effects on humans; in reality, different humans can be positively affected by social media (e.g. if it provides supportive online communities, or provides them with useful information). In another experiment, Lehman shows that this can be alleviated, as the LM can ask the agent (in a simulated transcript) about how social media makes them feel, so it can account for social media’s contributions to certain users’ well-being.
Why does it matter?
Machine learning systems are being deployed in many domains, and future systems will bring AI into even more domains. These systems should be trained in such a way that (a) human welfare (and not some unrelated metric) is improved and (b) is computationally feasible and tractable. Notions like machine love may bring us closer to (a); optimizing for richer notions of human flourishing will probably be better than optimizing for click-through rate, engagement with problematic media, or facial recognition accuracy on a biased dataset. There is still much work to be done in this direction; Maslow’s hierarchy of needs is probably not the best framework to understand human flourishing, and Fromm’s framework may not be the best for understanding love.
Moreover, (b) is going to be difficult for complex definitions of human flourishing. Supporting human flourishing is much more difficult than optimizing for engagement. The latter can be defined fairly well with one or a few numerical measurements, while the former is harder to define, measure, and improve. Even if we develop good methods of optimizing for some notion of machine love, such optimization can come with unintended impacts; for instance, optimizing for certain definitions of machine love may lead to over-paternalistic ML systems that exert too much control over the humans that they should care for. Much future work is required to overcome these limitations and difficulties, but such work may transform machine learning systems from narrow-minded metric optimizers to benevolent partners of humanity.
Author Q&A with Joel Lehman
Q: As you discuss in the paper, the idea of “human flourishing” isn’t very well-defined, but (broad question) how do you think about it?
A: Well — it’s notoriously difficult to definitively pin down. You can peruse the SEP to walk down the common philosophical positions (like hedonism or informed-desire-satisfaction) and clever (and intriguingly-named) thought experiments that highlight each position’s limitations (like the orphan monk or the grass-counter). Positive psychology also studies well-being, more from the lens of what distinct factors contribute to well-being, like the Ryff Scale (comprising self-acceptance, positive relationships, autonomy, environmental mastery, meaning and purpose, and personal growth). I think there’s something to each of these views, and that in absence of a conclusive definition, it’d be better to measure and/or encourage flourishing through a composite or individualized view.
Overall I’m skeptical that well-being/flourishing, as a byproduct of evolution, culture, and personal reasoning and values, can be boiled down to a single dimension, that any current theory provides the last word, or that different people with different minds must necessarily flourish in substantially similar ways. In some ways, I think flourishing could be seen as a hard-exploration reinforcement learning problem each of us face, although there may be many common elements shared across humanity. So I’m excited by the potential of LMs that have been trained across diverse human experience to assist us in that individualized exploration and help us to reflect and understand ourselves more deeply.
Q: An exciting connection you note in section 4.1 on Care is if LMs can make coarse estimates of growth and distinguish between addiction and flourishing, we might be able to bring this commonsense knowledge about growth into large-scale technological systems like recommendation systems. Especially with present worries about Tiktok being used as a “weapon against the west” and other discourse about the nefarious use of social media, how do you think about the dual-use capacities for this ability?
A: I do very much worry about how language models will be applied to influence humans. On the one hand, given that current objectives (like engagement) often bring about manipulation as an unintended side-effect, I feel somewhat less conflicted about trying to plot out a positive course for enhancing flourishing through ML with better objectives. On the other hand, I do worry whether this kind of work necessarily enables more nuanced ability for bad actors to directly manipulate humans.
Zooming out, there’s a kind of paralysis one can get into when trying to anticipate the Nth order effects of one’s research. It’s especially difficult for more blue-sky research (such as this paper). I do believe scientists bear moral responsibility for their work, and also that we as a society need to think more deeply about the unsolved alignment problem between science and society. But it’s tremendously difficult in a deep sense: Open-ended systems evolve in highly non-linear ways, and principles for how to search safely through such spaces are non-obvious (at least to me).
I think it is a topic worthy of much more study — the tension between creativity and control in open-ended search (e.g. science, law, market economies, our individual lives) more or less is the abstract cause of many difficult societal problems; and common positions, like axiomatic optimism or pessimism, both seem anti-scientific to me.
Q: I think your note at the end of 4.2 that the simulation of affect is unnecessary to meet the ends of machine love. I wonder whether, if you take prioritizing “real” relationships with other human beings to be an important part of human flourishing, you could even go farther and view affect as (at least a bit) detrimental to machine love’s ends—here, I’m thinking about recent stories we’ve seen concerning people confessing their love for chatbots.
A: I’m much more comfortable with aiming ML at encouraging human relationships with other humans (and animals), rather than having ML simulate affect or relationships. I can admit partly that it’s a personal bias in that it seems unnecessary and morally complex to wade into that terrain — and perhaps many lonely people may benefit in the short-term from talking with friendly non-judgmental AI companions.
However, I’m very concerned about the unforeseen implications of large-scale intimacy with machines. In general, the further and faster we move out of distribution from what relationships have evolutionarily and culturally meant to us, the more I worry we’ll later regret lacking the wisdom to approach with extreme caution the displacement of something critically important to our species (e.g. loving relationships with other humans), with something half-baked. See Chesterton’s fence, or the harms from our fast adoption of social media.
Q: I thought it was interesting that LMs have basic attachment theory competence. Can you speak to any other findings that were particularly surprising or notable to you?
A: Interestingly, it never occurred to me during the work to simply prompt a LM directly about Fromm’s four principles of loving action (which provide the proof-of-concept approach to machine love in the paper). Hat tip to @dribnet for reaching out and sharing that Bing chat could talk about Fromm with some capability; and it does seem like ChatGPT can as well. So it would be interesting to explore whether such models could more directly evaluate whether actions violate or support Erich Fromm’s principles, or whether in general they can directly implement other proposals for machine love or measuring flourishing. That I didn’t consider this possibility when writing the paper likely reflects that even though I’ve been working hands-on with LMs for several years, that it is hard to internalize how quickly their capabilities have improved.
Q: I really appreciated the integration of ideas like Fromm’s loving action. What immediate thoughts/plans do you have for extending this work, and what other (even unrelated) avenues do you see for integrating humanistic knowledge into ML?
A: I’m still considering where to go next with this work (and welcome suggestions, criticisms, and collaborations).
On the more general point of integrating humanistic knowledge into ML, I believe this is a very promising area and of critical importance. In my opinion, ML researchers and research institutions have not done a great job of recognizing how enormous their impact on society is becoming. In my experience, while there is often an acknowledgement of the need for interdisciplinary collaboration and even at times explicit calls for social scientists to assist making AI more safe, in practice at many of the major research labs there are not strong and focused collaborations between ML researchers and philosophers, political scientists, psychologists, or economists.
But I view such deep collaborations as essential to helping to anticipate and remedy the ways ML will continue to sweep through society. Progress in LMs call into question key assumptions underlying our institutions (for example, that human autonomy and epistemic rationality are girders to democracy), and we may need to buttress our institutions or cautiously reimagine them through the lens of this new technology, for our institutions (like news media, education, legal system, and politics) to maintain coherence. Organizations like the Center for Humane Technology, the Collective Intelligence Project, the AI Objectives Institute, and I’m sure many others, are attempting to help tackle this important problem.
On a more scientific note, I think ML may offer intriguing ways to ground out theories within philosophy, psychotherapy, economics, and other humanistic endeavors, which may otherwise sometimes operate at the level of unrealistic axioms (e.g. economics) or nebulously-specified and therefore hard to test or refute theories (e.g. psychotherapy or moral philosophy).
Q: Is love all you need? :)
A: I’ll be sappy first. I do believe that with a deep-enough conception of love, one committed to the practical skills of supporting each others’ aspirations and growth, in the limit, it might be all we need. There’d be a lot to unpack there, in terms of why and how.
But from a practical angle — no, sadly, I do not believe that love is enough. The intersections of game theory, politics, economics, and evolutionary psychology, seem to me to have somewhat entrapped us into problematic dynamics as a species, and I worry about our potential extinction in the decades to come. Beyond love, we need much critical thought, imagination, science, art, courage, and will-power for humanity to continue to blossom and flourish into future centuries.
New from the Gradient
Joe Edelman: Meaning-Aligned AI
Ed Grefenstette: Language, Semantics, Cohere
Other Things That Caught Our Eyes
News
The exciting new AI transforming search — and maybe everything — explained “Generative AI is here. Let’s hope we’re ready. The world’s first generative AI-powered search engine is here, and it’s in love with you. Or it thinks you’re kind of like Hitler.”
Salesforce to add ChatGPT to Slack as part of OpenAI partnership “Salesforce Inc (CRM.N) said on Tuesday it was working with ChatGPT creator OpenAI to add the chatbot sensation to its collaboration software Slack, as well as bring generative artificial intelligence to its business software generally.”
Meta must face trial over AI trade secrets, judge says “Meta Platforms Inc (META.O) lost a bid on Monday to end a lawsuit in Boston federal court claiming it stole confidential information from artificial-intelligence startup Neural Magic Inc.”
SETI: How AI-boosted satellites, robots could help search for life on other planets “AI-infused algorithms developed to find signs of life in extreme terrestrial environments could help robotic rovers sent to other planets search for signs of alien life, scientists suggested in new research published in Nature Astronomy on Monday.”
An AI ‘Sexbot’ Fed My Hidden Desires—and Then Refused to Play “My introduction into the world of AI chatbot technology began as the most magical things in life do: with a generous mix of horniness and curiosity. Early this year, as ChatGPT entered the general lexicon, a smattering of bot-related headlines began appearing in my social media newsfeeds.”
French surveillance system for Olympics moves forward, despite civil rights campaign “A controversial video surveillance system cleared a legislative hurdle Wednesday to be used during the 2024 Paris Summer Olympics amid opposition from left-leaning French politicians and digital rights NGOs, who argue it infringes upon privacy standards.”
Papers
Daniel: “Permutation Equivariant Neural Functionals” is a really neat recent paper. Neural Functional Networks (NFNs) can process the weights or gradients of other networks—this has a wide range of applications. NFNs can extract information from implicit neural representations (INRs) of data to do “image classification,” edit INR weights to produce visual changes, predict the test accuracy of convolutional neural network classifiers, and more. The design of this paper’s neural functionals accounts for the permutation symmetries in deep feedforward network weights. I thought one of the really neat applications of NFNs was predicting “winning ticket” masks (in reference to the Lottery Ticket Hypothesis) from initialization. There is a lot of potential for weight-space tasks, and I’d be excited to see these methods improved and applied to Transformers, ResNets, and more.
Tanmay: I found this paper on scaling down visual-language models to learn better representation for robotics tasks really interesting. Their model, named “Voltron”, uses a masked autoencoder and a transformer model to strike a balance between learning high level and low level features within an image. Their method does not need robot data, and can therefore be used zero shot on downstream tasks such as predicting a task’s running success rate.
Derek: “Dropout Reduces Underfitting” by Liu et al. is really cool. It closely investigates the motivation of using dropout to reduce overfitting, and shows that you can use this 10+ year-old tool for the opposite goal of reducing underfitting. Motivated by analyzing mini-batch gradients at different times in training, the authors show that only using dropout in early stages of training can decrease the final training loss. This is especially useful in the underfitting regime, which is relevant for smaller models, large datasets, and/or large amounts of data augmentation.
Tweets
Closing Thoughts
Have something to say about this edition’s topics? Shoot us an email at editor@thegradient.pub and we will consider sharing the most interesting thoughts from readers to share in the next newsletter! For feedback, you can also reach Daniel directly at dbashir@hmc.edu or on Twitter. If you enjoyed this newsletter, consider donating to The Gradient via a Substack subscription, which helps keep this grad-student / volunteer-run project afloat. Thanks for reading the latest Update from the Gradient!
Thanks, I think I could have more carefully worded that claim—Tencent’s own use of WeChat data is known (+ Beijing govt at least plans to take shares in Tencent). Some pointers
- https://qz.com/974408/tencents-wechat-gives-it-an-advantage-in-the-global-artificial-intelligence-race
- https://www.reuters.com/world/china/china-uses-ai-software-improve-its-surveillance-capabilities-2022-04-08/
Could you share your references for this claim? "It is well known that apps like WeChat and other data collection sources have allowed state-backed Chinese companies to develop powerful supervised learning algorithms."