The Gradient Update #15: Timnit Gebru Starts Her Own AI Research Center, DeepMind's Improvement Upon GPT-3
In which we discuss Timnit Gebru's new Distributed AI Research Institute and DeepMind's work on Scaling Language Models: Methods, Analysis, & Insights from Training Gopher
Welcome to the 15th update from the Gradient! If you were referred by a friend, subscribe and follow us on Twitter!
News Highlight: Ex-Googler Timnit Gebru Starts Her Own AI Research Center
SUMMARY
Last year, Timnit Gebru, a leader in examining the societal impacts of technology, tweeted that she had been fired from Google. A year later, Gebru has formed her own AI research center. Dubbed Distributed Artificial Intelligence Research (DAIR), the institute will, according to its founder, dare to ask questions about the responsible use of artificial intelligence that other companies won't dabble in. “Instead of fighting from the inside, I want to show a model for an independent institution with a different set of incentive structures,” says Gebru.
BACKGROUND
The paper that Gebru was working on before her exit from Google discussed ethical issues raised by recent advances in foundation models. An internal review at the company had found the contents of the paper to be objectionable and Gebru was told to either remove her name from the paper or retract the study entirely. Following this episode, Gebru tweeted that she had been fired by Jeff Dean, Senior Fellow & Senior Vice President at Google AI. While Google maintained that she was not fired but had resigned, Gebru's terse tweet courted substantial support from the research community and notable firms at large, including Microsoft and Nvidia. Many asserted that Google had maligned its own reputation in the field of natural language research—a field that is a bedrock of Google's developmental roadmap for AI research according to CEO Sundar Pichai. “You’re not going to have papers that make the company happy all the time and don’t point out problems,” Gebru said in an interview. “I felt like we were being censored and thought this had implications for all of ethical AI research."
WHY IT MATTERS
The fallout at Google sheds light on the inherent conflicts within tech companies and their employees who investigate the implications and impacts of the technology the company seeks to generate revenue from. Gebru's exit from Google and the fact that these are crucial foundational years for AI-based language models, will be the centerpieces for her new research center. She affirms that DAIR's studies will be free from bias and the workers will be unencumbered in their approach to investigating AI-based technologies. DAIR will also aim to be more inclusive than most AI labs and touch upon uncharted research areas. The center’s first work debuted at NeurIPS this year where it used satellite imagery of South Africa to investigate the impacts of apartheid in land use and distribution in the country today.
Editor Comments
Justin: It’s hard to articulate how inspired I felt the first time I saw Dr. Gebru, delivering a keynote address on the harms that Artificial Intelligence is wrecking on Palestistians in Gaza and the West Bank. To see that now, after the numerous ham-fisted attempts by corporate overlords to silence and stop her research, she has a platform to deliver groundbreaking research free from interference, fills me with optimism and anticipation.
Andrey: I think this is really cool news! This initiative is exciting for a few reasons. Firstly, the idea of having an AI ethics research institute not funded by industry makes a lot of sense, since the incentives of industry are often in opposition to those of AI ethics research. Secondly, the idea of a distributed AI research institute is really interesting. With COVID (mostly) behind us, it’s become pretty clear that while not ideal, it is entirely possible for many AI researchers to collaborate remotely. Designing an institute around this idea has a lot of benefits in terms of enabling a larger variety of people to get involved. Excited to see where this goes!
Daniel: I’m excited about this for similar reasons to Andrey–the key reason I think AI ethics research in large companies doesn’t feel substantive is that there are conflicting incentives. I don’t think those conflicting incentives are necessarily a bad thing–a company needs to preserve its bottom line and if it provides a useful service to the world, then so be it–but that does have implications for how we should view its output in research areas like AI ethics and expect it to be affected by these incentives.
Paper Highlight: Scaling Language Models: Methods, Analysis, & Insights from Training Gopher
BACKGROUND
Since GPT-3 astonished many with its capabilities last year, a number of organizations and states have also invested into developing large, pre-trained language models. Dubbed “foundation models” by Stanford researchers, these models have drawn attention for their capabilities and impact, both in influencing research directions and their ethical/societal impacts. Organizations such as Anthropic have arisen to study the capabilities and limitations of these models. Unsurprisingly, DeepMind has also stepped into the scene. To contribute to the development and study of these large models, DeepMind released three papers: the Gopher paper, a study of ethical and social risks of large language models, and an investigation of a new architecture with better training efficiency.
SUMMARY
Using the autoregressive transformer architecture in Radford et al (2019) with some modifications, the DeepMind researchers trained 6 models of varying sizes--the largest, at 280 billion parameters, was named Gopher. They showed they could achieve near state of the art results in over 150 distinct tasks, and also compiled a “a holistic analysis of the training dataset and model’s behaviour, covering the intersection of model scale with bias and toxicity”.
They conclude the paper with two main points:
“The landscape of language technologies with general capabilities is progressing rapidly. Language models are a key driver of this progress, and we have shown that an emphasis on data quality and scale still yields interesting performance advances over existing work. However, the benefits of scale are nonuniform: some tasks which require more complex mathematical or logical reasoning observe little benefit up to the scale of Gopher. This may be an inherent property of the language modelling objective — it is hard to compress mathematics and easier to learn many associative facts about the world … Alongside the development of more powerful language models, we advocate broad development of analysis and interpretability tools to better understand model behaviour and fairness, both to guide mitigation of harms and to better inform the use of these models as a tool to scalably align artificial intelligence to societal benefit.”
At the same time, they also released Ethical and social risks of harm from Language Models, a lengthy analysis of issues that might arise from the use of these models, with the main analysis being a taxonomy summarizing these potential issues:
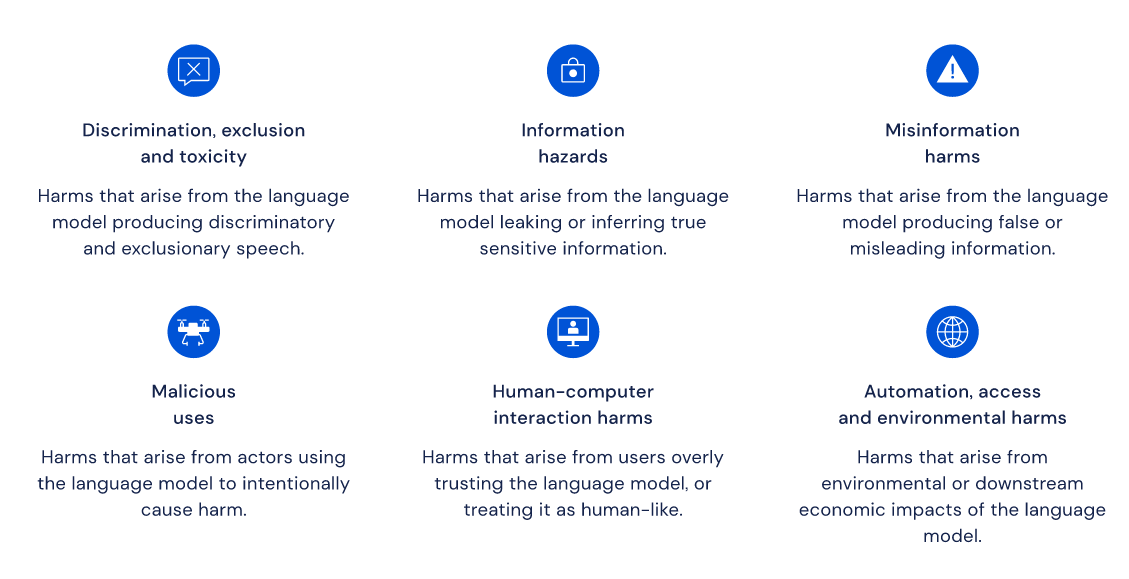
Their hope is that
“The taxonomy we present serves as a foundation for experts and wider public discourse to build a shared overview of ethical and social considerations on language models, make responsible decisions, and exchange approaches to dealing with the identified risks.”
WHY IT MATTERS
To the practitioner, these three papers present an excellent roadmap for building a large language model to support any reasonable language task. While a well articulated set of procedures for recreation is expected of the scientific method, the accompanying 64 page ethical study on the technology is largely unprecedented in our field. It is particularly relevant given the large shadow that still hangs over other industry giants for their role in undermining the research into the dangers of large language models. The presented taxonomy is the first of its kind for such models, and will hopefully make thinking through how to avoid these negative consequences from the use of these models.
Editor Comments
Justin: While I loved that the authors did attempt to address harmful biases learned by their models, I wish they chose a different approach than the overly broad “toxic”. There are all kinds of specific harms that these models can perpetuate like racism or sexual harasmment. By focusing on a specific disruptive behavior, there is an opportunity to catalogue the harms in an actionable way for the developers and maintainers of language models in the wild (production).
Andrey: Due to the importance of this class of models, I am glad to see more papers exploring their properties. There is a lot of analysis in these several works, and some interesting insights. While nothing too surprising was revealed, it’s still an excellent step forward in gaining better understanding and reasoning about where to go next from here.
New from the Gradient
Other Things That Caught Our Eyes
News
MIT robot could help people with limited mobility dress themselves "Robots have plenty of potential to help people with limited mobility, including models that could help the infirm put on clothes. That's a particularly challenging task, however, that requires dexterity, safety and speed."
FTC Signals It May Conduct Privacy, AI, & Civil Rights Rulemaking “The Federal Trade Commission announced Friday that it is considering using its rulemaking authority “to curb lax security practices, limit privacy abuses, and ensure that algorithmic decision-making does not result in unlawful discrimination.”
Toronto considers banning sidewalk robots “The Toronto City Council is considering banning mobile robots from sidewalks and bike paths. The provision was originally put forward by the Toronto Accessibility Advisory Committee.”
Facebook AI’s FLAVA Foundational Model Tackles Vision, Language, and Vision & Language Tasks All at Once “Current state-of-the-art vision and vision-and-language models are generally either cross-modal (contrastive) or multi-modal (with earlier fusion) and tend to target specific modalities or tasks.”
U.N. chief urges action on 'killer robots' as Geneva talks open “United Nations Secretary-General Antonio Guterres called on Monday for new rules covering the use of autonomous weapons as a key meeting on the issue opened in Geneva. Negotiators at the U.N.”
Papers
Tell me why! -- Explanations support learning of relational and causal structure - “Explanations play a considerable role in human learning, especially in areas that remain major challenges for AI -- forming abstractions, and learning about the relational and causal structure of the world. Here, we explore whether reinforcement learning agents might likewise benefit from explanations. We outline a family of relational tasks that involve selecting an object that is the odd one out in a set (i.e., unique along one of many possible feature dimensions). Odd-one-out tasks require agents to reason over multi-dimensional relationships among a set of objects. We show that agents do not learn these tasks well from reward alone, but achieve >90% performance when they are also trained to generate language explaining object properties or why a choice is correct or incorrect. …”
Reduced, Reused and Recycled: The Life of a Dataset in Machine Learning Research - “Despite the foundational role of benchmarking practices in this field, relatively little attention has been paid to the dynamics of benchmark dataset use and reuse, within or across machine learning subcommunities. In this paper, we dig into these dynamics. We study how dataset usage patterns differ across machine learning subcommunities and across time from 2015-2020. We find increasing concentration on fewer and fewer datasets within task communities, significant adoption of datasets from other tasks, and concentration across the field on datasets that have been introduced by researchers situated within a small number of elite institutions. Our results have implications for scientific evaluation, AI ethics, and equity/access within the field.”
Tweets

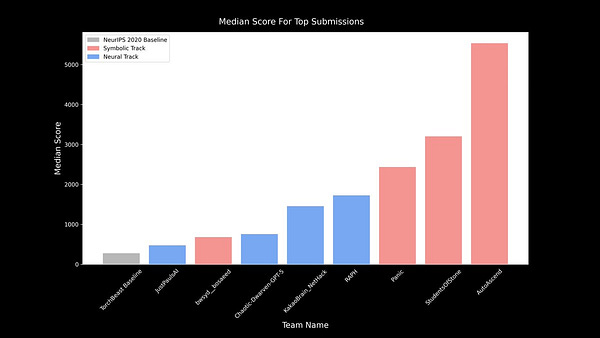


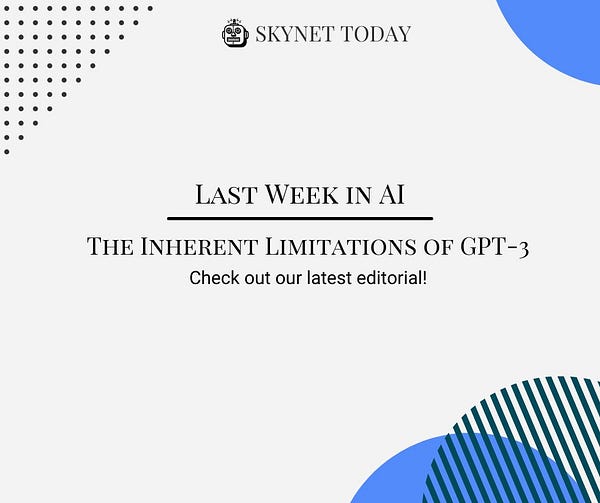
Closing Thoughts
Have something to say about this edition’s topics? Shoot us an email at gradientpub@gmail.com and we will consider sharing the most interesting thoughts from readers to share in the next newsletter! If you enjoyed this piece, consider donating to The Gradient via a Substack subscription, which helps keep this grad-student / volunteer-run project afloat. hanks for reading the latest Update from the Gradient!