Gradient Update #14: Israel’s Surveillance of Palestinians, Natural Adversarial Objects
In which we discuss Israel's newly revealed extensive surveillance program in Palestine and the new Natural Adversarial Objects dataset
Welcome to the 14th update from the Gradient! If you were referred by a friend, subscribe and follow us on Twitter!
News Highlight: Israel’s Surveillance of Palestinians: Elaborate, Clandestine, and Incentivized
SUMMARY
Recent interviews of Israeli soldiers have shed light upon the broad surveillance of Palestinians by the Israeli military. According to estimates, thousands of Palestinians, including children, women, and the elderly have been photographed under this program. Sourced through soldiers who are incentivized to photograph Palestinians, the pictures and videos amassed are uploaded to a database that categorizes the subject in one of three categories (or 'severities'): to be detained, arrested immediately, or allowed to pass. This profiling has been ongoing for the past couple of years. And unlike border checks, this profiling is clandestine; much of it happens without notification to the local populace.
BACKGROUND
While the political situation between Israel and Palestine is well-known, Israel’s thorough and surreptitious profiling of Palestinian citizens has remained in the shadows. The surveillance is carried out using a smartphone technology dubbed Blue Wolf, which uses a database to categorize Palestinian citizens based on their 'danger' to the Israeli settlers and the military force. According to a former soldier who was interviewed by The Post and Breaking the Silence, Blue Wolf’s database is a subset of the Wolf Pack database, which contains profiles of virtually every Palestinian in the West Bank. It includes photographs of the individuals, their family histories, education, and a security rating for each person.
To build Blue Wolf, the Israeli government incentivized soldiers to photograph Palestinian citizens in the West Bank, particularly Judea, Samaria, and Hebron. Army units across the West Bank would compete for prizes, such as a night off, given to those who took the most photographs, former soldiers said. For some soldiers, taking the pictures was a daily mission that often lasted close to eight hours. Each unit would take hundreds of photos each week--one former soldier said their unit was expected to take at least 1,500.
In personal accounts and interviews, these soldiers spoke of the trauma of photographing the Palestinians without their consent. One former soldier said that while Palestinian children tended to pose for the photographs, but the elderly—particularly older women—would often resist.
When inquired about the surveillance program, the Israel Defense Forces (IDF) said that such “routine security operations” were “part of the fight against terrorism and the efforts to improve the quality of life for the Palestinian population in Judea and Samaria.”
WHY DOES IT MATTER?
Accounts of Blue Wolf come at a time when there is substantial debate surrounding the use of AI systems in profiling, monitoring, and surveilling citizens in the West. Shortly after the Black Lives Matter Movement picked up pace after the murder of George Floyd, IBM, Microsoft, and Amazon momentarily discontinued the purveyance of their facial recognition systems to the police forces until thorough and fair legislation is formulated around the technology. A couple of weeks back, surprisingly, even Facebook announced that it will be disabling its facial recognition system.
Israel’s use of surveillance and facial recognition appears to be among the most elaborate deployments of such technology by a country seeking to control a subject population, according to experts with the digital civil rights organization AccessNow. The IDF's take on BlueWolf and the stark distinction between the allowance of such technologies depending on the geopolitical region that they are deployed in shed light on the fact that for many, freedom and privacy are mere foreign concepts.
Paper Highlight: Natural Adversarial Objects
BACKGROUND
One common benchmark for measuring the performance of object recognition is MSCOCO, released by Microsoft with the “goal of advancing the state-of-the-art in object recognition by placing the question of object recognition in the context of the broader question of scene understanding.” In parallel, researchers have demonstrated across domains that large machine learning models often fall victim to adversarial attacks as well as struggle to perform on out-of-distribution data sets. To help remediate those two problems, researchers from Scale AI have released the Natural Adversarial Objects (NAO) dataset. NAO is similar to MSCOCO, diverging to include examples that test the limits, robustness, and generalizability of models trained on MSCOCO.
SUMMARY
“The goal of NAO is to benchmark the worst-case performance of state-of-the-art object detection models, while requiring that examples included in the benchmark are unmodified and naturally occurring in the real world”. After creating NAO, the researchers compared nearly a dozen state of the art models trained on MSCOCO and evaluated on NAO for their ability to generalize. They concluded that there are numerous “blind-spots” in MSCOCO and demonstrated that models trained on it are “overly sensitive to local texture but insensitive to background change”.
EDITOR REMARKS:
Justin: The most remarkable thing to me are the details that went into creating the datasets. At a time when the reproducibility of machine learning models is constantly being challenged, these procedures provide a clear procedure to not only recreate NAO but provide a roadmap for other researchers to develop their own domain-specific adversarial datasets.
Andrey: More and more AI research these days is focused on pointing out issues and limitations with existing research methodologies and benchmarks. It used to be that reviewers would be more negative towards such work, but more recently it has become more appreciated and even popular. I am a fan of this trend and hope it remains a constant for the future of AI research.
New from the Gradient
Miles Brundage on AI Misuse and Trustworthy AI
Jeffrey Ding on China's AI Dream, the AI 'Arms Race', and AI as a General Purpose Technology
Other Things That Caught Our Eyes
News
AI Will Create 97 Million Jobs, But Workers Don’t Have the Skills Required (Yet) - Artificial intelligence technologies have reduced repetitive work and enhanced work efficiency, and as a result, almost every industry in the world is planning to leverage AI or has already implemented it in their business.
OpenAI makes GPT-3 generally available through its API - Starting today, any developer in a supported country can sign up to begin integrating the model with their app or service.
Europe’s AI laws will cost companies a small fortune – but the payoff is trust - Hear from CIOs, CTOs, and other C-level and senior execs on data and AI strategies at the Future of Work Summit this January 12, 2022. Learn more Artificial intelligence isn’t tomorrow’s technology — it’s already here. Now too is the legislation proposing to regulate it.
Clearview AI told to stop processing UK data as ICO warns of possible fine - Controversial facial recognition company Clearview AI is facing a potential fine in the UK.
Europe’s AI Act falls far short on protecting fundamental rights, civil society groups warn - Civil society has been poring over the detail of the European Commission’s proposal for a risk-based framework for regulating applications of artificial intelligence which was proposed by the EU’s executive back in April.
Papers
Florence: A New Foundation Model for Computer Vision “... While existing vision foundation models such as CLIP, ALIGN, and Wu Dao 2.0 focus mainly on mapping images and textual representations to a cross-modal shared representation, we introduce a new computer vision foundation model, Florence, to expand the representations from coarse (scene) to fine (object), from static (images) to dynamic (videos), and from RGB to multiple modalities (caption, depth). By incorporating universal visual-language representations from Web-scale image-text data, our Florence model can be easily adapted for various computer vision tasks, such as classification, retrieval, object detection, VQA, image caption, video retrieval and action recognition. …”
Acquisition of Chess Knowledge in AlphaZero “What is learned by sophisticated neural network agents such as AlphaZero? ... In this work we provide evidence that human knowledge is acquired by the AlphaZero neural network as it trains on the game of chess. By probing for a broad range of human chess concepts we show when and where these concepts are represented in the AlphaZero network. We also provide a behavioural analysis focusing on opening play, including qualitative analysis from chess Grandmaster Vladimir Kramnik. Finally, we carry out a preliminary investigation looking at the low-level details of AlphaZero's representations, and make the resulting behavioural and representational analyses available online.”
A Survey of Generalisation in Deep Reinforcement Learning “The study of generalisation in deep Reinforcement Learning (RL) aims to produce RL algorithms whose policies generalise well to novel unseen situations at deployment time, avoiding overfitting to their training environments. ... This survey is an overview of this nascent field. We provide a unifying formalism and terminology for discussing different generalisation problems, building upon previous works. We go on to categorise existing benchmarks for generalisation, as well as current methods for tackling the generalisation problem. Finally, we provide a critical discussion of the current state of the field, including recommendations for future work. ...”
A Systematic Investigation of Commonsense Understanding in Large Language Models “Large language models have shown impressive performance on many natural language processing (NLP) tasks in a zero-shot setting. We ask whether these models exhibit commonsense understanding -- a critical component of NLP applications -- by evaluating models against four commonsense benchmarks. We find that the impressive zero-shot performance of large language models is mostly due to existence of dataset bias in our benchmarks. We also show that the zero-shot performance is sensitive to the choice of hyper-parameters and similarity of the benchmark to the pre-training datasets. Moreover, we did not observe substantial improvements when evaluating models in a few-shot setting. Finally, in contrast to previous work, we find that leveraging explicit commonsense knowledge does not yield substantial improvement.”
Tweets

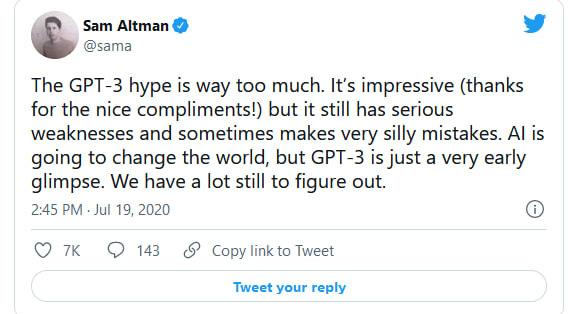

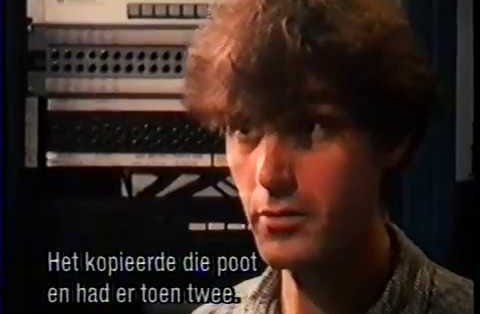
Closing Thoughts
Have something to say about this edition’s topics? Shoot us an email at gradientpub@gmail.com and we will consider sharing the most interesting thoughts from readers to share in the next newsletter! If you enjoyed this piece, consider donating to The Gradient via a Substack subscription, which helps keep this grad-student / volunteer-run project afloat. Thanks for reading the latest Update from the Gradient!