Update #71: Neuralink's First Human Trial and Controlling Language Model Hallucinations
Noland Arbaugh plays chess with his mind, and researchers find training strategies that reduce language model hallucinations.
Welcome to the 71st update from the Gradient! If you’re new and like what you see, subscribe and follow us on Twitter :) You’ll need to view this post on Substack to see the full newsletter!
We’re recruiting editors! If you’re interested in helping us edit essays for our magazine, reach out to editor@thegradient.pub.
Want to write with us? Send a pitch using this form.
News Highlight: Neuralink's First Human Trial: Mind-Control Chess with Brain Chip N1
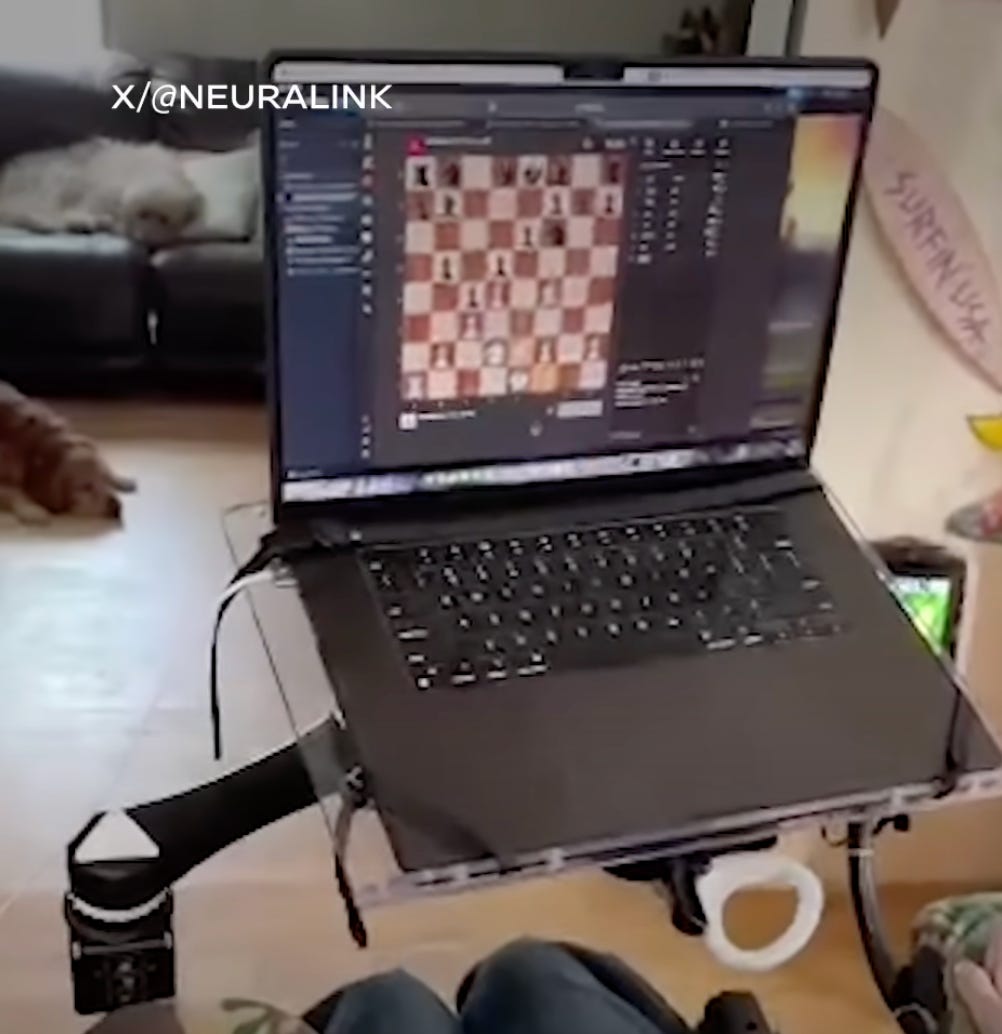
Summary
Neuralink, Elon Musk's brain-chip startup, showcased its first human trial with patient Noland Arbaugh, who played chess using his mind after being implanted with the company's brain-computer interface technology.
Overview
The human brain, home to around 86 billion neurons, is a marvel of nature. Every action we take, every thought we have, is the result of tiny electrical impulses generated and transmitted at incredible speeds from one neuron to another. Harnessing this complex neural activity, Neuralink's latest technology has offered a new lease on life to Noland Arbaugh, the first patient to get a cybernetic implant from Neuralink. Noland, a 29-year-old Arizona native and former student-athlete at Texas A&M, became quadriplegic after a diving accident in 2016. In a recent livestream on X, Noland is seen moving the cursor on a computer to play chess and the game Civilization VI without using any physical tool.
In January 2024, Elon Musk revealed that Neuralink had implanted its brain chip, called N1, in a human for the first time after receiving approval from the Food and Drug Administration (FDA) in May 2023. The implantation procedure, performed by a specialized surgical robot, involved embedding the device and its 64 ultra-thin flexible threads, containing 1,024 electrodes, directly into a region of the brain that controls movement intention (source: Neuralink). These electrodes record neural activity and transmit signals wirelessly to an app that decodes movement intention. The surgical robot is also equipped with five camera systems and utilizes optical coherence tomography (OCT) for noninvasive imaging of brain tissue. It employs a needle as fine as a human hair for precise insertion (source: Neuralink).
This device, called Telepathy, has the potential to significantly enhance the bandwidth of brain-machine communication, as it records from a larger number of neurons compared to Blackrock Neurotech's Brain-computer interfaces (BCIs), which are the only other single-neuron recording systems that have been implanted long-term in humans (source: Nature). While some users have had multiple Blackrock devices implanted, Neuralink's technology stands out due to the flexibility of its threads. Furthermore, Arbaugh reported a smooth post-surgery experience, being discharged from the hospital a day later with no cognitive impairments.
Controlling a computer cursor with only thoughts isn't a new milestone for BCIs; a similar achievement was accomplished with an older brain chip implanted in a human in 2004 (source: Practical Neurology, Wall Street Journal). However, the older chip required wired connections through the skin for data transmission, whereas Neuralink's device communicates wirelessly. Neuralink is among several companies in the brain-computer interface space, including Synchron, which has a stent-like device implanted in the jugular vein atop a patient's brain, and Precision Neuroscience, which has temporarily implanted its microelectrode array in six patients for data collection. In another study published in Nature in 2023, researchers from the Ecole Polytechnique Fédérale de Lausanne (EPFL) successfully implanted electronic devices into the brain and spinal cord of a paralyzed man, enabling wireless communication between the two. This fully implanted system links cortical signals to the spinal cord regions involved in walking, enabling natural control over leg movements for standing, walking, climbing stairs, and traversing complex terrains.
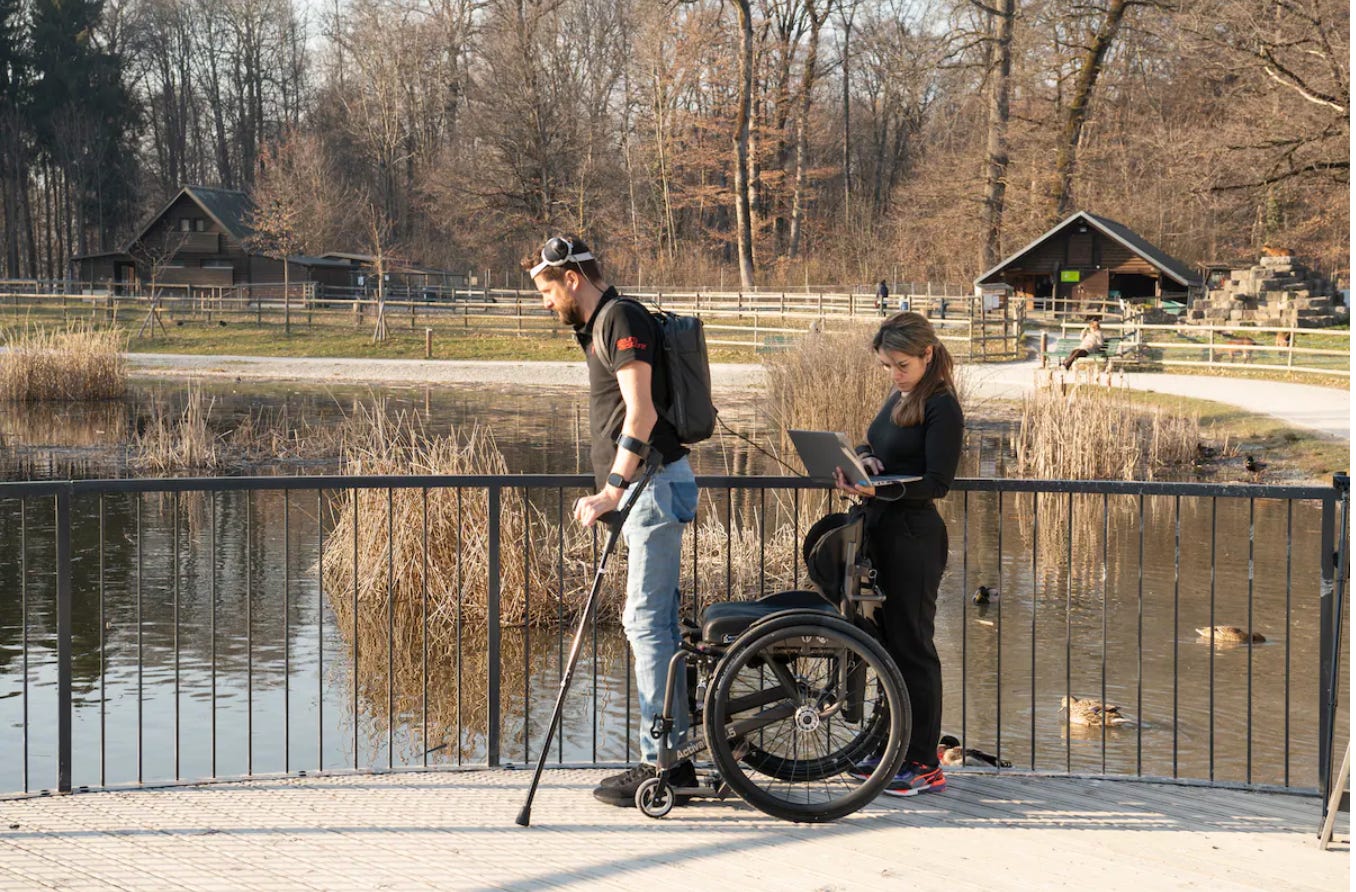
Our Take
Brain-computer interfaces (BCIs) offer exciting possibilities for enhancing human capabilities and addressing neurological conditions. However, their adoption faces several challenges, including ensuring safety and long-term effectiveness, addressing ethical concerns, and achieving minimal damage while implantation in the human body. Transparency in research and trials is crucial to address these challenges and build trust in the technology.
Neuralink's first human trial is a significant step forward in neurotechnology, but it also raises important concerns. The lack of transparency regarding trial details, such as the number of subjects and specific outcomes being assessed, is troubling. This is on top of Neuralink's prior experiments with monkeys that have sparked controversy, with reports indicating that some animals had to be euthanized due to complications, including brain bleeds, bloody diarrhea and more (source: The Verge).
Further the developed brain-machine interaction (BCI) system works in one direction -- brain to machine. Bidirectional implants that also provide feedback to users will be key to Neuralink’s vision to assist individuals with paralysis in regaining communication abilities and to restoring motor, sensory, and visual functions, and treating neurological disorders. However, its path to approval and market entry seems long and challenging, potentially taking significantly longer than the commoditization of its one-way implant, which is already far. Elon Musk's ultimate vision in his own words is “to achieve a symbiosis with artificial intelligence”, allowing us to merge with AI and stay relevant as it advances. However, I believe that there are many more layers to this objective that extend beyond the technical aspects. For instance, the question of whether individuals with such implants should compete with those without is complex and involved.
Lastly, over time, a device implanted in the brain could face issues like electrode degradation, tissue scarring, or biofouling, which can affect its functionality and safety. Continuous monitoring is essential to detect and address such problems, ensuring the device remains effective and does not harm the user over a long horizon. As technology evolves, it will be crucial to balance innovation and responsibility, ensuring that it serves the greater good and addresses the needs of those it intends to help.
- Sharut
Research Highlight: Unfamiliar Finetuning Examples Control How Language Models Hallucinate
Summary
Researchers at UC Berkeley and Google’s DeepMind unveiled a new paper on Large Language Model (LLM) hallucinations and training strategies designed to reduce the number of hallucinations made by language models. The team found that as LLM inputs become more unfamiliar (relative to the training distribution) their outputs tend to default to a “hedged position.” Through experimentation, they discover this hedged position is determined by the form and structure of other unfamiliar examples introduced in the LLM’s fine tuning stage. By strategically controlling the label distributions of these unfamiliar fine-tuned examples, the authors show how they can reduce LLM’s hallucination rate on a variety of tasks, such as multiple choice Q&A and biography generation.
Overview
To understand how models hallucinate, the authors begin by fine-tuning Llama2-7B on a question answering dataset (TriviaQA). As seen below in the chart on the right, for queries whose subjects are not well-represented in the pretraining corpus, the false answer rate increases to over 60% for LLMs trained using standard SFT.
Further investigation into the false positives revealed that the models do not randomly hallucinate, but tend to default towards a singular hedged position. The authors described the hedged position as an intelligent guess that arrives from minimizing the aggregate finetuning loss over all the unfamiliar examples. It follows that a model trained with SFT would have a default hedge position that is similar to the fine tuned ground truth distribution.
They empirically demonstrate how the default hedge position mimics the ground truth of the finetuning distribution by training two question answering (QA) models. The first model, shown at the top of the below chart, has uniformly distributed labels for all samples (familiar and unfamiliar). The second model has biased the unfamiliar samples labels towards either B or C.
For the authors’ hypothesis to hold, their first model, trained on data with uniformly distributed labels, would have a default hedge position that is uniformly random (¼ = 25%) since the labels for unfamiliar examples are uniformly distributed. Similarly, for the second hypothesis, the unfamiliar samples, which have been biased towards a label distribution that was uniformly distributed over two labels, B and C. Those two labels would each have a probability of 50% while the others would be 0%. As we will see below, for each model they trained, they observed that the default hedge position mimicked the distribution of the unfamiliar fine tuned examples as they hypothesized.
As seen above, the first model (top row) which was fine tuned on unbiased label distributions, performs best with familiar examples (Test Example NLL is approximately 0) while with unfamiliar samples performs randomly (p = 25%). This is in contrast to the second model (second row) which, when faced with unfamiliar inputs, mimics the label distribution of unfamiliar fine tuned examples ( P(B or C) = 100%, P(B) = P(C) = 50% )). The authors then go on to demonstrate that by creating a potential fifth answer, E, to represent a model’s uncertainty about the true answer for unfamiliar samples, they could fine tune to guide the model’s behavior for other underrepresented samples.
While these experiments showed evidence that the distribution of underrepresented data in fine tuning guided a model's behavior when faced with uncertainty, they don’t provide a scalable path towards reducing hallucinations on their own. This is because it requires a large corpus of human label examples that are designed to capture the expected uncertainty of a model for each finetuning task. That task grows increasingly complex as the domain of knowledge covered models varies with more models, data, and different tasks.
To find a path towards scalably reducing LLM hallucinations, the authors turn to reinforcement learning via conservative reward models (RM). The authors’ key insight is that while reward model hallucinations may be inevitable, through strategic control of finetuned data, they can control how reward models hallucinate and reduce the number of hallucinations. Specifically, their reward function is designed to underestimate the reward when faced with underrepresented inputs. The researchers demonstrate the value of this reward function by running experiments comparing SFT, standard reinforcement learning (RL), and RL with a conservative reward model. They found for both Biography and Plot Generation, the conservative reward model produced the smallest number of hallucinations. The number of hallucinations is inferred from the number of false facts, which was 2-5x lower for the conservative RM compared to the other two models for the most unfamiliar examples (where freq ~= 0).
Our Take
Model hallucinations, alternatively known as mistakes, errors, uh ohs, or boo boos are a particularly prevalent problem for large generative models across domains (text, image, video, audio). Solving for them remains one of the largest hurdles towards both the commercial success of large AI companies as well as the public's trust in their model’s quality and reliability. While this body of work is focused primarily on understanding the development and mitigation of hallucinations in LLM’s the findings could likely generalize across domains. I am very interested in the future of this space and to see whether or not there are further domain generalizations can be achieved and if the learnings here can be adopted across domains
-Justin
New from the Gradient
Kate Park: Data Engines for Vision and Language
Ben Wellington: ML for Finance and Storytelling through Data
Other Things That Caught Our Eyes
News
Reddit’s Sale of User Data for AI Training Draws FTC Inquiry
Reddit, ahead of its IPO, revealed that it could generate $203 million in revenue over the next few years by licensing user posts to Google and other companies for AI projects. However, the US Federal Trade Commission (FTC) has raised concerns about the sale, licensing, or sharing of user-generated content for AI training. The FTC has the authority to penalize companies engaged in unfair or deceptive trade practices. Other platforms, such as StackOverflow and the Associated Press, have also entered into similar data licensing agreements. Reddit stated that it does not believe it has engaged in any unfair practices, but acknowledged that dealing with a government inquiry can be costly and time-consuming.
Too Much Trust in AI Poses Unexpected Threats to the Scientific Process
While ML is helping researchers in various scientific fields make new discoveries and predictions, relying too heavily on these systems can pose risks. Some of these risks include the replication and amplification of human biases, the environmental costs of running complex AI models, and the tendency for humans to automatically trust and attribute authority to machines—a paper published in Nature highlights the potential effects of trusting AI technology too much and calls for caution in its use.
xAI open sources base model of Grok, but without any training code
The xAI team has open sourced the base code of Grok on GitHub, but the training code is not included. Grok, which has 314 billion parameters, was previously released as a chatbot accessible to Premium+ users of X / Twitter. Other companies, such as Perplexity, are already planning to use Grok in their solutions. The model is licensed under Apache License 2.0, allowing for commercial use.
Using AI to spot edible mushrooms could kill you
This article highlights a recent study that found AI algorithms were not accurate enough to reliably distinguish between edible and poisonous mushrooms. The study tested several popular mushroom identification apps and found that they often misidentified poisonous mushrooms as safe to eat. The author warns against relying solely on AI for mushroom identification and emphasizes the importance of consulting with an expert or using multiple sources of information.
AI researchers now reviewing their peers with AI assistance
Researchers from Stanford University, NEC Labs America, and UC Santa Barbara have used generative AI to analyze peer reviews of papers submitted to leading AI conferences, aiming to evaluate the impact of LLMs on peer reviews. The authors found a small but consistent increase in apparent LLM usage for reviews submitted close to the deadline. They also identified that LLMs tend to use certain adjectives more frequently than human authors, allowing them to identify reviews where LLM assistance is likely. The study suggests that between 6.5% and 16.9% of peer reviews could have been substantially modified by LLMs. The authors argue for more transparency in the use of LLMs and caution against the potential homogenization effect and biases introduced by AI feedback.
Ubisoft debuts NEO NPC AI prototypes
Ubisoft has showcased its NEO NPC AI prototypes at GDC 2024, demonstrating the potential of AI in creating non-player characters (NPCs) with more depth and responsiveness. The project combines AI responses with narrative backstories and prompts to make the NPCs feel more lifelike. The demos featured interactions with NPCs named Bloom and Iron, where players could build relationships, ask questions, and receive scripted information relevant to the game. The objective is to enhance narrative immersion and allow players to level up their relationship with the NPCs. Ubisoft believes that these smarter NPCs have the potential to create more immersive worlds and emergent stories.
Guiding Principles for the Church’s Use of Artificial Intelligence
The Church of Jesus Christ of Latter-day Saints has issued guiding principles for the use of AI in its work. The principles emphasize the Church's commitment to using AI in ways that “support… the connection between God and His children.” Transparency, privacy, security, and accountability are also key considerations in the Church's use of AI. The Church sees several opportunities for AI, including in family history work, automating processes, and language translation. Elder Gong, a member of the Quorum of the Twelve Apostles, expressed optimism about the Church's ability to use AI wisely and effectively while protecting against falsehoods and deception.
Denmark is partnering with NVIDIA to establish a national center for AI innovation that will house one of the world's most powerful AI supercomputers. The collaboration, led by the Novo Nordisk Foundation and the Export and Investment Fund of Denmark, aims to accelerate research and innovation in fields such as healthcare, life sciences, and the green transition. The supercomputer, named Gefion, will be a large-scale NVIDIA DGX SuperPOD powered by NVIDIA H100 Tensor Core GPUs and interconnected using NVIDIA Quantum-2 InfiniBand networking. It will enable researchers in Denmark to pursue large-scale projects and engage with expert teams at NVIDIA to co-develop solutions to complex problems. The Danish Centre for AI Innovation is expected to be ready for pilot projects by the end of the year.
Apple is adding to its arsenal of AI startups with a little-known Canadian firm
Apple has acquired DarwinAI, a Canadian AI startup based in Waterloo, Ontario. The company has developed AI technology for visually inspecting components during manufacturing and has also worked on making AI systems smaller and faster. The acquisition is expected to enhance Apple's supply chain efficiency. DarwinAI's co-founder, Alexander Wong, has joined Apple to lead its AI group.
An AI-driven “factory of drugs” claims to have hit a big milestone
An AI-driven "factory of drugs" called Insilico Medicine claims to have achieved a significant milestone in drug discovery. The company used AI software to determine both the target inside a cell to interact with and the chemical structure of the drug. This approach led to the synthesis and testing of a drug candidate in just 18 months, demonstrating that AI can accelerate the drug discovery process. However, despite the hype around AI in biotech, many startups in the field have struggled to deliver on their promises. The high cost and failure rate of drug development remain significant challenges.
Chinese platforms are cracking down on influencers selling AI lessons
Chinese social platforms WeChat and Douyin have started cracking down on influencers who sell AI lessons after receiving complaints from students about the superficiality of the courses. Influencers like Li Yizhou, who have no background in AI, have been selling entry-level and advanced AI courses for a significant profit. However, buyers have reported that the courses lacked actual content and were focused on urging people to pay for more expensive courses. In addition, it was difficult for buyers to get refunds. As a result, the platforms have removed all classes by these influencers and suspended their accounts.
States are racing ahead of Congress to regulate deepfakes
At least 15 states in the US have passed laws concerning deepfakes, with a focus on two main applications: politics and pornography. These laws aim to address the growing number of fraud and abuse cases involving deepfakes, such as AI-generated porn of celebrities and scammers impersonating loved ones. However, the patchwork implementation of state laws leaves loopholes and puts the burden on individuals to keep up with the latest laws in their state. Congress is attempting to pass federal deepfake legislation to create a standard across all states, but bipartisan support is uncertain. Proposed federal bills target deepfake porn and seek to create new protections for "likenesses" in general. However, there are First Amendment challenges and concerns about the ability to detect and filter deepfake content. Striking the right balance between regulation and freedom of speech will be challenging, and enforcing these laws will be difficult as deepfake technology becomes more accessible.
OpenAI’s chatbot store is filling up with spam
The GPT Store, OpenAI's marketplace for custom chatbots powered by its generative AI models, is facing issues with spam and copyright infringement. The store, which has grown rapidly and currently has around 3 million GPTs, contains GPTs that generate art in the style of Disney and Marvel properties, as well as GPTs that claim to bypass AI content detection tools. There are also GPTs that promote academic dishonesty by suggesting they can evade plagiarism detectors. Additionally, the store features GPTs that impersonate people or organizations without their consent. OpenAI's moderation efforts seem to be lacking, and the company may face legal issues due to copyright infringement and impersonation. The GPT Store's current state raises concerns about the quality and adherence to OpenAI's policies.
Can AI Replace Human Research Participants? These Scientists See Risks
A new review paper accepted for the Association for Computing Machinery's Conference on Human Factors in Computing Systems (CHI) explores the idea of using LLMs to replace human participants in scientific studies. The paper cites several studies and commercial products that propose using LLMs to stand in for human research subjects or analyze research outcomes. The potential benefits of using AI to synthesize data include increased speed, reduced costs, avoidance of risks to participants, and augmented diversity. However, the paper's authors argue that these methods conflict with the central values of research involving human participants. Skeptics also worry that AI-synthesized data may not accurately represent human experiences and could produce biased or scientifically shoddy results.
Stability AI CEO resigns to ‘pursue decentralized AI’
Emad Mostaque, the CEO of Stability AI, has resigned from his position to pursue decentralized AI. Mostaque will also step down from his position on the board of directors. Stability AI has appointed two interim co-CEOs, Shan Shan Wong and Christian Laforte, while they search for a permanent CEO. Mostaque's departure comes after other key developers resigned from the company.
Nearly 4,000 celebrities found to be victims of deepfake pornography
An investigation by Channel 4 News has revealed that nearly 4,000 celebrities, including 255 British individuals, have become victims of deepfake pornography. The investigation analyzed the five most visited deepfake websites and found that these sites received 100 million views in just three months. Deepfake pornography involves using artificial intelligence to superimpose the faces of famous individuals onto pornographic material. The Online Safety Act, which came into effect in the UK on January 31, 2023, makes sharing such imagery without consent illegal, but the creation of the content is not. The broadcasting watchdog Ofcom is currently consulting on how the act will be enforced and applied.
Papers
Daniel: A lot of raving about Sakana AI’s Evolutionary Model Merge paper, which is indeed really cool. Evolutionary optimization / search is another direction for scaling AI systems that holds a lot of promise, so it’s neat to see this work.
Two neat papers on systematic biases in transformer-based models from different angles, one general and one specific. This paper finds a general bias in transformers to learn low-sensitivity functions—lower than alternative architectures—which correlates with robustness. This second paper finds that protein language models are systematically biased towards certain species, in that the likelihood of protein sequences from these species are higher than ones from other species. This turns out to be a result of unequal species representation in protein sequence databases.
Finally, in the interest of the fundamental limitations of next-token prediction as a paradigm, this paper treats the question carefully by separating two phases of next-token prediction and interrogating an assumption made by popular criticism of the paradigm.
Closing Thoughts
Have something to say about this edition’s topics? Shoot us an email at editor@thegradient.pub and we will consider sharing the most interesting thoughts from readers to share in the next newsletter! For feedback, you can also reach Daniel directly at dbashir@hmc.edu or on Twitter. If you enjoyed this newsletter, consider donating to The Gradient via a Substack subscription, which helps keep this grad-student / volunteer-run project afloat. Thanks for reading the latest Update from the Gradient!
I don't think playing to Neuralink's PR orchestra helps readers when you state "the patient... played chess using his mind after being implanted with the company's brain-computer interface..." The patient could play chess before the implant operation - and, yes, he'd definitely have to use his mind. The implant only estimated where his visual attention was with respect to a fixed screen image, and then an external computer directed a cursor to that point. This only achieves the same effect as non-invasive eyeball tracking. There is an immense amount of work still to do - and proper multi-patient trials over several years - to gather enough evidence to convince regulators and achieve mainstream medical acceptance. Successful health outcomes relate to patient mobility, occupational and self-care improvement, not how cool the tech is from exaggeration and distortion of coarse neural correlates.