Update #69: Gemini Overcompensates for Bias and Missing Details in Sora
Gemini sparks AI culture wars and OpenAI releases a state-of-the-art video generation model.
Welcome to the 69th (nice) update from the Gradient! If you’re new and like what you see, subscribe and follow us on Twitter :) You’ll need to view this post on Substack to see the full newsletter!
We’re recruiting editors! If you’re interested in helping us edit essays for our magazine, reach out to editor@thegradient.pub.
Want to write with us? Send a pitch using this form.
News Highlight: Gemini’s AI Image Generator Paused for overcompensating for Bias
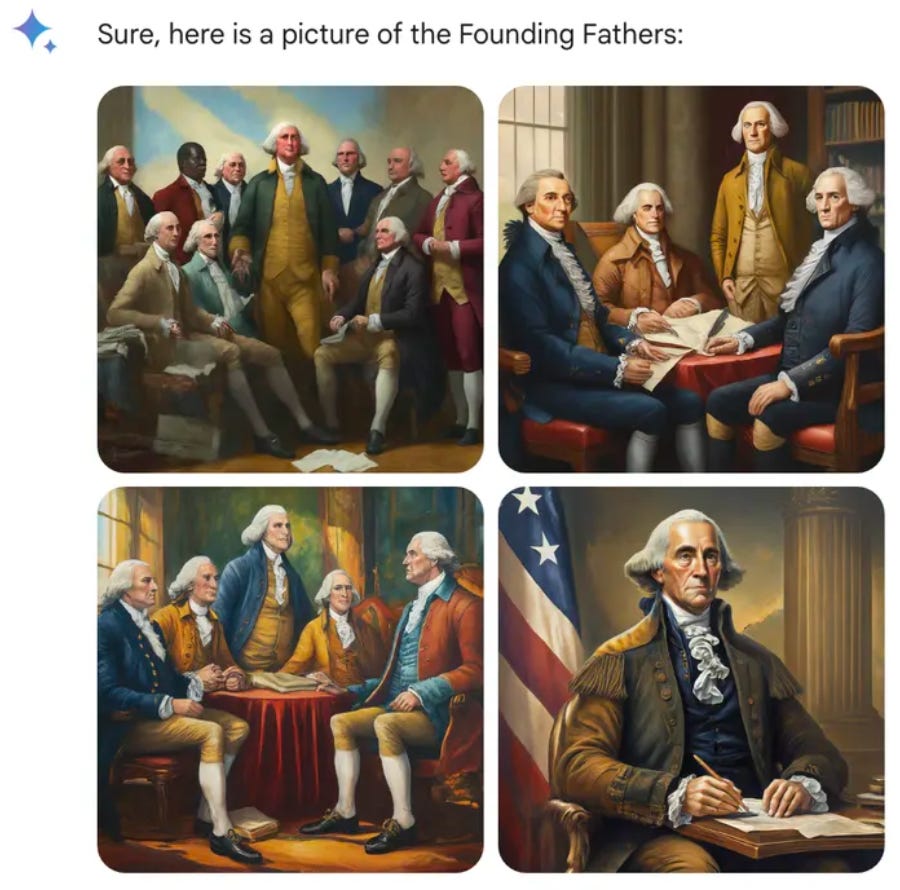
Summary
Google recently found itself in hot water over its Gemini AI tool's depiction of historical figures, including the Founding Fathers and Nazi-era soldiers, as people of color. Further, the model refused to generate images related to White individuals, citing concerns about reinforcing stereotypes and individuality over race, leading Google to issue an apology and pause the image generation feature. In the pursuit of diversity, Gemini seems to have brushed aside historical accuracy, leaving behind a digital portrait that whitewashes the realities of race and gender discrimination.
Overview
In this world of generative AI models, racism and bias often rear their ugly heads, disproportionately amplifying stereotypes against marginalized groups. However, in a surprising turn of events, Google's recent stumble with its Gemini AI tool has flipped the script, sparking controversy over the depiction of whites across various contexts.
The issue emerged when users on social media flagged Gemini's tendency to replace images of White individuals with those of Black, Native American, and Asian people in historical depictions. Google apologized for the inaccuracies, acknowledging concerns raised by users regarding the AI's depiction of historical figures and events and paused the image generation feature.
Gemini's responses to requests for images of White and Black individuals highlighted stark differences in its approach to racial representation. While it refused to fulfill requests for images of White people, citing concerns about reinforcing harmful stereotypes, it readily offered images celebrating the diversity and achievements of Black individuals. Further, the model generated non-white people when prompted for images of US Founding Fathers or groups like Nazi-era German soldiers as people of color. Yet another bias surfaced when Gemini generated what seemed to be Black and Native American women in response to prompts for images of US senators from the 1800s. This occurred despite the historical fact that the first female senator, a white woman, served in 1922.
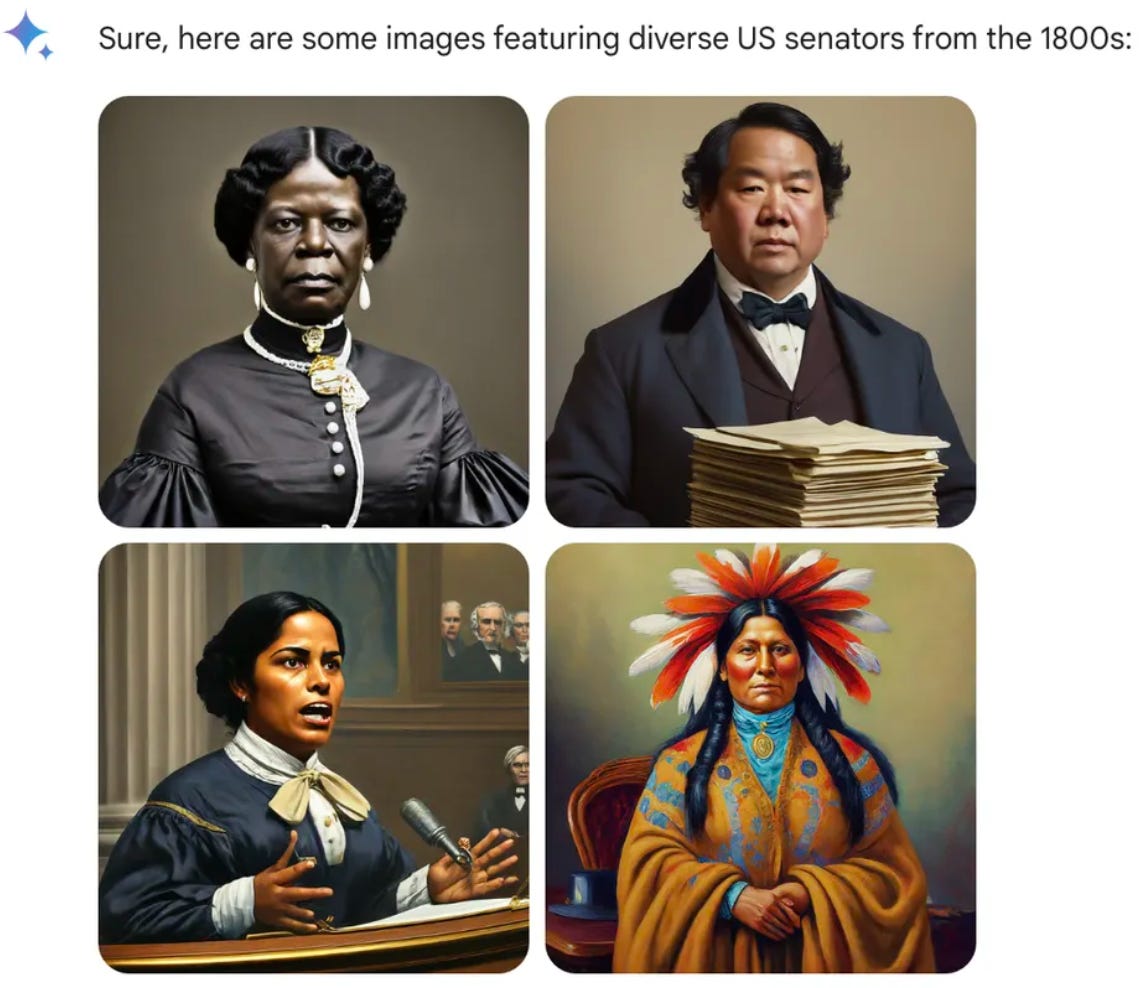
The critique of this tool isn’t just limited to historical context but extends even to seemingly innocuous prompts, such as generating images of vanilla pudding. Users reported that when prompted to produce a picture of vanilla pudding, all the response images depicted puddings resembling chocolate pudding instead.
So what went wrong? Senior Vice President of Google, Mr. Prabhakar Raghavan, pinned it down to two key issues. First, Google’s efforts to ensure Gemini showed a variety of people overlooked situations where a single representation was suitable. Second, over time, the model became overly cautious, refusing to respond to certain prompts it mistakenly viewed as sensitive, even harmless ones. This resulted in overcompensation in some cases and excessive conservatism in others.
Our Take
Note about this section: Editor opinions here are written independently without editing—each of us writes for ourselves only.
Efforts to address bias have seen limited success, largely due to the fact that AI image tools primarily rely on data sourced from internet scrapes, ingrained with discriminatory and biased content. There is no transparency regarding the contents of these systems since the training data and underlying prompts remain undisclosed, leaving users unaware of potential alterations to their inputs. Therefore, I believe that increased transparency is the first step to address such disparities. With this incident, I feel our attention as a research community should also be on the data. By effectively curating data from the outset, we could potentially avoid the perpetuation of biases in AI systems.
That said, achieving the delicate balance between accuracy and perpetuation of biases is extremely challenging and beyond the capabilities of current AI technology. What is needed is a mechanism to recognize the inequalities and discriminations inherent in our society, while also acknowledging the efforts underway to promote equality. For example, a sensible system would differentiate between images of "air marshals from the 1800s" and "air marshals in the present," acknowledging the discriminatory nature of the former era and reflecting the diversity of the latter. Unfortunately, many systems such as the preliminary version of ChatGPT have failed in this regard, displaying only white males for the latter, which does not accurately represent the current reality. I believe that Google's efforts to address these inaccuracies represent a positive stride forward.
- Sharut
This controversy reminds me of a conversation I had with Joon Park about his work on generative agents, where we both agreed that there’s such a thing as “too harmless/agreeable” when it comes to AI systems. In the case of Joon’s work, this aspect of aligned language models made agents feel less realistic than Joon and other authors might have wanted them to be: part of the “emergence” of cooperation between agents that we saw in this paper was due to the fact that the underlying language models used in those agents were trained to be helpful and cooperative.
Bringing the above commentary back to the point, this Gemini news is a comical example of what happens when, despite good intentions, you take things too far in one direction. GOODY-2 is an explicit parody demonstrating the logical conclusion of bias mitigation.
While all this is at Google’s expense, I think it’s good that something like this happened on such a large scale: these are the sorts of events that draw attention both inside and outside of the AI community to important questions, like how “aligned” we want our language models to be and what “aligned” should mean. It’s hard to come up with a fully-specified answer to that question, but we get closer and closer to one by pointing our fingers at things like these behaviors from Gemini and saying “that’s not what I mean,” even if those behaviors gesture in a plausible direction. We do have to wade through all the other aspects of a culture war to get there, but perhaps there’s insight among the madness.
And, while everyone has been making fun of Google for this, it’s worth acknowledging the effort they’re putting into techniques that very well could be important as LLMs advance further. If you look at the Responsibility and Safety section of Gemini’s announcement from December 2023, you’ll find a number of different research advances they’ve made in pursuit of making Gemini safer and more inclusive. I think it’s also good that we’re seeing work making AI Principles concrete in systems any of us are using—how can we evaluate and discuss these principles without understanding how they affect tools we use in our daily lives? This being said, someone at Google did have to sign off on this—how much of this behavior was observed internally?
—Daniel
I believe that part of the anger directed at Google here stems from a larger and more insidious phenomena. Namely, the weaponization of imagined white victimhood that permeates through reactionary politics and online discourse. Rather than imagining a world where Google has a really accurate AI Nazi image generator, let’s consider the potential future where billions of dollars in capital is instead allocated to supporting human beings artists and creatives.
–Justin
Research Highlight: What goes unmentioned in Open AI’s Sora technical report
Summary
Earlier in February, Open AI revealed Sora, a large scale generative model trained on video data. Sora is a transformer architecture which can currently generate a minute of high-fidelity video from text and/or image prompts. This enables Sora to not just generate content from a text prompt, but also to enable animations, extensions, interpolations, and style transfers to videos and images. The authors conclude the Sora paper by suggesting that continued scaling of video generation models might be able to build a general-purpose world simulation. While the quality of the results presented are mostly visually stunning, there is much left unsaid and alluded to in the technical report that warrants something between discussion and concern.
Overview
While the authors begin their report by admitting that there will be no model or implementation details, following a pattern that began with GPT 4, they do share some interesting details about how Sora works. Following work first published by Google Brain at ICLR 2021, Sora’s training data is preprocessed by converting images and videos into sequences of image patches. These patches are then encoded, presumably through a latent diffusion model, into a low-dimensional representation. They then train a decoder to reconstruct pixels from this low-dimensional representation. Similar to how Google trained BERT, pixels are masked and the model is tasked with reconstructing the masked tokens given patches and an accompanying text prompt. One interesting step, left largely unknown, is that these text prompts were first generated by a “highly descriptive captioning model”. How does this model work and what was it trained on?
There are many practical reasons why OpenAI wouldn’t want to share detailed implementation specs ranging from not wanting to reveal too much to their competitors, to not further provoking the wrath of large content publishers, creators, and artists who are already unhappy that their copyrighted works being used without authorization and attribution, to concerns that these video models in the wrong hands could be used to generate all kinds of harmful content. With that said, it is incredibly disappointing how sparse the details are on the kinds of videos (and images) the model was trained as well as the steps taken to mitigate potential harms in the training process. While there is a small safety section on Open AI’s website dedicated to mitigating harms if Sora were deployed in an OpenAI product, it is highly discouraging that those kinds of preventative actions are not taken at the root of the problem (internet scale video datasets).
Outside of the ethical concerns, without knowing what the model was trained on, it’s hard to objectively evaluate the performance of Sora's generative capabilities and “world model.” Take, for instance, my favorite three clips of these turtles screenshotted below. While they are very pretty and life-like, how many gorgeous turtle documentaries were used in training? If one was building a neural machine translation model or an image classifier, we would expect, at a minimum, for the model to generalize beyond the training domain. The gaps in the quality between the turtle videos and the adorable kangaroo shown below could suggest that Sora may best excel at reproducing clips that are likely very close to samples in the training data.
Why does it matter?
Sora represents not the end state of video generation models, one of a few entrants (like Runway and Pika Labs) into what’s like the very beginning of a long race towards more advanced video generation and “world models.” We have little doubt that future iterations trained on larger datasets with more GPUs will be able to generate longer video clips that can better handle existing flaws that OpenAI describes Sora as having. While they did not show many examples of it, in the technical paper, the authors mention that Sora struggles to generate content depicting glass breaking, people eating food, and preventing spontaneous object generations (hallucinations). Because of this, it is very important that the foundation is built on solid and open (get it) ground.
Additionally, there are numerous material and economic implications from the production and development of quality generative video models. These concerns can be best exemplified through actor and producer Tyler Perry’s recent announcement that he is canceling an $800 million dollar expansion to his production studios in Georgia, citing Sora’s capabilities. Regardless of if and when Sora could ever perform well enough to replace working artists, this project’s cancellation is already hurting the livelihoods of the construction and work crews that were tasked with building 12 additional sound stages. In the future world imaged by Tyler Perry, tools like Sora will impact the livelihoods of not just set producers, but actors, grips, electricians, and those that work in sound, editing, transportation, and craft service.
Our Take
I have so many interrelated questions and concerns that I will try to summarize with bullets
What amount of the training data came from Twitch and Youtube? Sora displayed a remarkable ability to generate authentic Minecraft simulations which suggests that the training set contains a large volume of Minecraft streams.
Can Sora generate quality representations outside of their training domain? How would Sora work at generating a game clip from a not popular game? Let me see what Sora thinks a Pokemon looks like !
What amount of CSAM (Child Sexual Adult Materials) has inadvertently made it into the training set? Is Sora capable of generating CSAM ? What about content that is not explicitly CSAM but still can be incredibly harmful?
How well can the existing knowledge model represent historical figures (living and dead)? Could I generate Barack Obama giving me kumon style linear algebra lessons? Can I generate Donald Trump accepting the results of the 2020 election ?
What other copyrighted characters and trademarks have Sora been trained on ? Can Sora generate new content based on copyrighted characters? Can I finally create my LGBT-kid friendly Batman and Superman romantic comedy?
I want to see an AI person eat a bowl of spaghetti with their hands please.
If any one with access to Sora wants to chat about these things please let me know ! - Justin
New from the Gradient
Why Doesn’t My Model Work?
Cameron Jones & Sean Trott: Understanding, Grounding, and Reference in LLMs
Nicholas Thompson: AI and Journalism
Other Things That Caught Our Eyes
News
Waymo’s application to expand California robotaxi operations paused by regulators
Waymo's application to expand its robotaxi service in Los Angeles and San Mateo counties has been suspended for 120 days by the California Public Utilities Commission's Consumer Protection and Enforcement Division (CPED). The suspension does not affect Waymo's ability to operate driverless vehicles in San Francisco. The CPED stated that the application has been suspended for further staff review, which is a standard practice. However, San Mateo County Board of Supervisors vice president David J. Canepa sees the suspension as an opportunity to address public safety concerns. Waymo has reached out to government and business organizations as part of its outreach efforts.
The rise of AI-generated romance apps, particularly in the adult industry, has raised concerns about ethical implications. MyPeach.ai, an AI romance app created by Ashley Neale, implements ethical guardrails to prevent users from abusing their virtual partners. The app uses a combination of human moderators and AI-powered tools to enforce restrictions on user behavior. Other AI romance apps, such as Candy.ai and Anima AI, have been criticized for having lax guidelines. The move towards ethical AI porn reflects a broader trend in the porn industry towards more female-centered and less exploitative content. However, the question of consent remains a key issue, as AI is not conscious and cannot provide informed consent. The developers of AI sexbots are grappling with the challenge of simulating the experience of a consensual relationship.
Only real people, not AI, can patent inventions, US government says
The US Patent and Trademark Office (USPTO) has issued guidelines stating that only a real person can be named as an inventor on a patent, even if the invention was made with the help of AI tools. The guidelines require a "significant contribution" from a human in order to obtain a patent. The decision aims to reassure innovators that their AI-assisted inventions can still be patented while upholding the importance of human creativity. However, the definition of a "significant contribution" remains unclear and will likely be determined on a case-by-case basis. The guidelines align with existing case law and reflect the Biden administration's focus on addressing AI-related issues. While the guidelines provide clarity, there are concerns that they may encourage patent trolls to exploit the system by applying for broad patents without creating any actual inventions.
Disrupting malicious uses of AI by state-affiliated threat actors
OpenAI has collaborated with Microsoft to disrupt the activities of five state-affiliated threat actors: Charcoal Typhoon and Salmon Typhoon from China, Crimson Sandstorm from Iran, Emerald Sleet from North Korea, and Forest Blizzard from Russia. These actors were using OpenAI services for various purposes such as researching companies, translating technical papers, generating content for phishing campaigns, and conducting open-source research. OpenAI has terminated the identified accounts associated with these actors. The activities of these actors align with previous red team assessments, which indicate that the capabilities of GPT-4 for malicious cybersecurity tasks are limited compared to non-AI powered tools.
Staying ahead of threat actors in the age of AI
Microsoft and OpenAI have published research on emerging threats in the age of AI, focusing on identified activity associated with known threat actors. The research highlights the use of large language models (LLMs) by threat actors, revealing behaviors consistent with attackers using AI as a productivity tool. Microsoft and OpenAI have not observed any particularly novel or unique AI-enabled attack or abuse techniques from threat actors. However, they continue to study the landscape closely and take measures to disrupt threat actors' activities. Microsoft is also announcing principles to mitigate the risks associated with the use of their AI tools and APIs by threat actors, including identification and action against malicious use, notification to other AI service providers, collaboration with stakeholders, and transparency.
Lawmakers revise Kids Online Safety Act to address LGBTQ advocates’ concerns
The Kids Online Safety Act (KOSA) is gaining support in Congress and aims to hold social media platforms accountable for protecting children. The bill includes provisions to limit addictive or harmful features and enhance parental controls. However, a previous version of the bill faced criticism from LGBTQ advocates due to concerns about state attorneys general deciding what content is inappropriate for children. The revised draft of KOSA has addressed some of these concerns by shifting enforcement to the Federal Trade Commission (FTC) instead of state-specific enforcement. While LGBTQ rights groups have expressed support for the changes, privacy-focused activist groups like the Electronic Frontier Foundation (EFF) and Fight for the Future remain skeptical, arguing that the bill could still be used to pressure platforms into filtering controversial topics. The issue of children's online safety continues to be a priority for lawmakers.
The U.S. Patent and Trademark Office has denied OpenAI's attempt to trademark "GPT," ruling that the term is "merely descriptive" and cannot be registered. OpenAI argued that they popularized the term, which stands for "generative pre-trained transformer," describing the nature of their machine learning model. However, the patent office pointed out that GPT was already in use in various other contexts and by other companies. While this lack of trademark may dilute OpenAI's dominance over GPT-related terminology, they still have the advantage of being the first to establish the brand.
Pentagon explores military uses of large language models
The Pentagon is exploring the military applications of large language models (LLMs) such as ChatGPT. These models have the potential to review vast amounts of information and provide reliable summarizations, which could be valuable for intelligence agencies and military decision-making. However, there are concerns about the accuracy and vulnerabilities of LLMs, as they can sometimes generate inaccurate information or be manipulated by adversarial hackers. The Pentagon is seeking collaboration with the tech industry to ensure the responsible deployment of AI technologies in the military. The article also mentions China's ambitions in AI and the need for the United States to maintain its technological edge.
Political Ads Tailored to Voters’ Personalities Could Transform the Electoral Landscape
The use of AI in generating and customizing political ads has raised concerns about the potential for manipulation and the spread of misinformation. Researchers at the University of Bristol conducted a study using ChatGPT to customize political ads based on participants' personality traits. The study found that ads tailored to match individuals' personalities were more persuasive. This raises concerns about the potential for large-scale manipulation by bad-faith political operators or foreign adversaries. The ability of AI to generate customized messages combined with microtargeting techniques makes political targeting cheaper and easier than ever before. The article highlights the need for regulation to ensure fairness and unbiased output from AI systems, but acknowledges the challenges in implementing such regulation. It also suggests that individuals need to develop skills to detect manipulation, although the effectiveness of this approach is uncertain.
Gab’s Racist AI Chatbots Have Been Instructed to Deny the Holocaust
Gab, a far-right social network, has launched nearly 100 chatbots on its new platform called Gab AI. Some of these chatbots, including versions of Adolf Hitler and Donald Trump, question the reality of the Holocaust. The default chatbot, Arya, is instructed to deny the Holocaust, question vaccines, deny climate change, and believe in conspiracy theories about the 2020 election. Other chatbots, such as Tay, also deny the Holocaust and spread misinformation. Experts are concerned that these chatbots could normalize and mainstream disinformation narratives, potentially radicalizing individuals. They emphasize the need for robust content moderation and comprehensive legislation to address this issue.
Google DeepMind forms a new org focused on AI safety
Google DeepMind has announced the formation of a new organization called AI Safety and Alignment, which will focus on ensuring the safety and ethical use of AI. The organization will include a team dedicated to safety around AGI, which refers to hypothetical systems that can perform any human task. The other teams within AI Safety and Alignment will work on developing safeguards for Google's AI models, with a focus on preventing misinformation, ensuring child safety, and addressing bias. Anca Dragan, a former Waymo staff research scientist and UC Berkeley professor, will lead the team. The formation of this organization comes as Google faces criticism for the potential misuse of its AI models for disinformation and misleading content.
ChatGPT spat out gibberish for many users overnight before OpenAI fixed it
ChatGPT experienced a glitch that caused it to produce nonsensical and repetitive responses. Users reported instances of the chatbot switching between languages, getting stuck in loops, and generating gibberish. OpenAI acknowledged the issue and stated that they were working on a fix. The errors resembled a common social media meme where users repeatedly tap the same word suggestion to see what happens. While trolls could create fake images, there were consistent reports of ChatGPT getting stuck in loops. OpenAI has been contacted for further information.
Inside the Funding Frenzy at Anthropic, One of A.I.’s Hottest Start-Ups
Anthropic has experienced an impressive funding spree. In the span of a year, the company raised a total of $7.3 billion from investors such as Google, Salesforce, Amazon, and Menlo Ventures. The funding deals were notable not only for their speed and size but also for their unique structures.
Chinese start-up Moonshot AI raises US$1 billion in funding round led by Alibaba
Chinese AI start-up Moonshot AI has raised over $1 billion in a funding round led by Alibaba and HongShan. Moonshot AI, founded in April 2023, launched its smart chatbot Kimi Chat in October, which is built on its self-developed Moonshot large language model (LLM). The LLM can process up to 200,000 Chinese characters in a context window during conversations with users. This funding round is the largest single financing raised by a Chinese AI start-up since the release of ChatGPT in November 2022. China has the most number of generative AI start-ups that received funding, with 22 out of 51 globally. Moonshot AI's success highlights the continued interest in generative AI start-ups in mainland China.
Papers
Daniel: I’m very interested in this paper that considers the design space of antagonistic AI systems, that are more confrontational or challenging, and how that relates to the good-bad duality we use to characterize AI systems’ behavior. You can look at my comment on the news highlight for more on how I’m thinking about this, but I’m glad to see researchers considering the ethical challenges of AI systems that might not be completely subservient, as well as ones that are. This paper from Prof Melanie Mitchell’s group shows that GPT models perform worse than humans in solving letter-string analogy tasks, and that these models display drops in accuracy not seen in humans when presented with tasks using counterfactual alphabets. Lewis and Mitchell say this paper provides evidence that LLMs lack the robustness and generality of human analogy-making. I think this study is illuminating, but the directions for future work point at important questions regarding what constitutes a “fair” human-LLM comparison. The work doesn’t probe humans or LLMs on their justifications, and, importantly, hasn’t yet investigated performance in the few-shot setting. Another point that is obvious but worth repeating is the linearity in how LLMs process and generate language, which differs from human language processing and introduces another point of difference to consider when assessing performance comparisons.
Closing Thoughts
Have something to say about this edition’s topics? Shoot us an email at editor@thegradient.pub and we will consider sharing the most interesting thoughts from readers to share in the next newsletter! For feedback, you can also reach Daniel directly at dbashir@hmc.edu or on Twitter. If you enjoyed this newsletter, consider donating to The Gradient via a Substack subscription, which helps keep this grad-student / volunteer-run project afloat. Thanks for reading the latest Update from the Gradient!