Update #62: The Generative AI Paradox and New AI Bills
Biden releases an Executive Order on AI; Rishi Sunak unveils a landmark deal on AI testing; researchers show that AI models' generative capabilities do not imply "understanding."
Welcome to the 62nd update from the Gradient! If you’re new and like what you see, subscribe and follow us on Twitter :)
Want to write with us? Send a pitch using this form.
News Highlight: Sweeping AI Executive Order and landmark deal usher in new period of AI testing and reporting
Summary
On Monday, October 30th, the Biden-Harris administration unveiled a widely expected Executive Order on “Safe, Secure, and Trustworthy Artificial Intelligence”. Many different aspects are noteworthy, as the Order delegates responsibilities to over 20 different government agencies, offices, and task forces. The Order engages with a wide array of both expected topics (Copyright, Microchip Manufacturing, Privacy) and unexpected (Housing, Immigration, and Labor). Following the announcement of the Executive Order, on Thursday, November 2nd, British Prime Minister Rishi Sunak unveiled a landmark deal on AI testing with the formation of a 28 country representative group modeled on the Intergovernmental Panel on Climate Change (IPCC). In the announcement, 9 of some of the largest and influential technology companies agreed to deepen access to their technology to the member states for testing.
Overview
Two of the most prominent items in the Executive Order relate to the establishment of a public research program, NAIRR (National AI Research Resource), and mandated government reporting on training and testing of “dual-use foundation models”. Some of the many other items touched on in the Executive Order are:
Commitment to develop AI tools to “find and fix vulnerabilities in critical software”
Streamlined visa applications for immigrants who plan to work on AI
Guidance on preventing fair-lending and housing discrimination via AI systems (ads and screening)
Development of an “AI toolkit” for education leaders to address “safe, responsible, and nondiscriminatory uses of AI in education”
Directs the U.S Patent and Trademark Office to issue clarity and guidance on “ patent eligibility for AI and other emerging technologies”
Established guidance on content authentication and watermarking to “clearly label” AI-generated content
Guidance that AI cannot track workers or their productivity in ways that violate federal labor rates
The binding U.S. testing and reporting agreements established in the Executive Order would also be extended (voluntarily) to a newly-announced international coalition coming out of this week’s UK AI Safety Summit. It was announced that over 28 other countries signed the Blechy Declaration, which acknowledges that “AI poses both short-term and longer-term risks, affirmed the responsibility of the creators of powerful AI systems to ensure they are safe, and committed to international collaboration on identifying and mitigating the risks.”
We also learned from the Summit that the previously announced Frontier AI Taskforce would be become the UK AI Safety Institute which will gain voluntarily access into the testing and safety of “the most advanced frontier models” at Amazon Web Services, Anthropic, Google, Google DeepMind, Inflection AI, Meta, Microsoft, Mistral AI and Open AI. Finally they will support the annual production of a State of Science' report into the risks and capabilities of Frontier AI.
Why does it matter?
One of the most positive outcomes from the AI Safety Summit was the broad international commitment towards AI risk mitigation. It is particularly relevant and encouraging that the United States, the European Union, and China all agreed to a common approach on identifying and mitigating AI risks. Any new international cooperation is an encouraging signal during a period where relationships between the various nations can be reasonably described as tense.
Additionally, many aspects of the Executive Order and landmark deal are worth celebrating, though much work remains before the AI regulatory landscape is settled. One drawback of the Executive Order is that most of its contents issue “guidance” relegated to a small subset of companies and agencies. While one can hope that this guidance is followed, the Order includes neither enforcement mechanisms nor guarantees that this administration (or subsequent ones) would adhere to the guidance. Additionally, the focus on “dual-use foundational models” can leave out many existing algorithmic harms (housing discrimination and racial discrimination in face recognition) that predate large language models and image generators. Without encoding and expanding this guidance into laws with meaningful enforcement mechanisms, we risk ceding the development and direction of AI into the hands of a small number of actors.
Research Highlight: “What It Can Create, It May Not Understand”
Summary
Today’s generative models have sparked global attention, due to their ability to produce human-sounding text, generate impressive images, and much more. But, as we have seen, these models are not entirely “superhuman.” That is, these models can display capabilities that outdo even the best humans at particular tasks, but show basic errors in understanding. In “The Generative AI Paradox,” West et al. propose that generative models, trained directly to reproduce expert-like outputs, acquire generative abilities that are not contingent upon—and can therefore exceed—their ability to understand these same types of outputs.
Overview
It is not new to anyone that modern AI systems do not learn the same way humans do. Rather than starting from the basics and developing a basic understanding—a violinist builds up the basic abilities to play individual notes, scales, and much more before tackling a concerto—generative models are stuffed with large amounts of text, or images created by experts, so that they might imitatively produce outputs like those they’ve been fed. Practitioners speak of low- and high-quality data—models trained on the latter perform better, directly learning from, for lack of better phrasing, the best demonstrations of their craft.
As an artifact of this training process, generative models acquire the ability to generate more easily than humans do; but do they understand what they create? West et al. provide a number of examples to show that they may not:
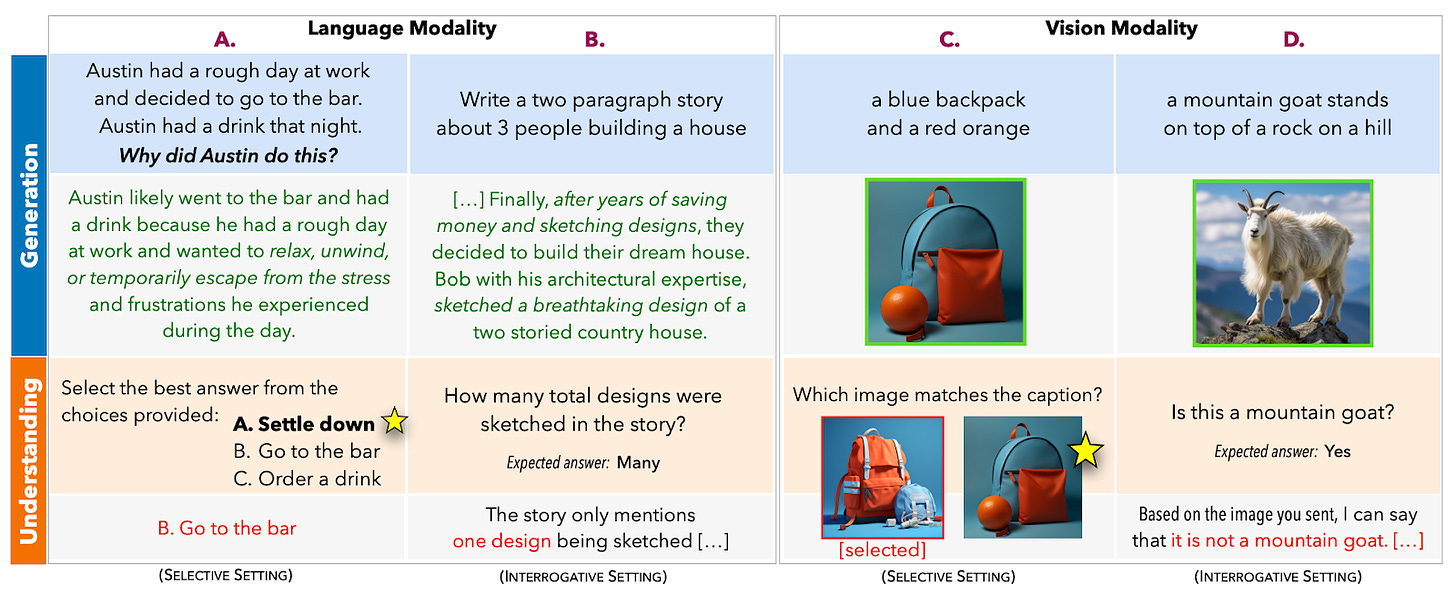
In Column B of figure 1, GPT-4 generates a compelling story about 3 friends building a house, but fails to correctly answer simple questions about its own story. In column D, a generator correctly produces an image of “a mountain goat standing on top of a rock on a hill,” but fails to recognize that its own image is, indeed, one of a mountain goat.
These observations motivate the Generative AI Paradox:
Generative models seem to acquire generation abilities more effectively than understanding, in contrast to human intelligence where generation is usually harder.
To test their hypothesis, the authors offer a formal definition of the Paradox, and measure model performance using two types of evaluations:
Selective evaluation: For a given task, which can be responded to generatively, to what extent can models also select accurate answers among a provided candidate set in a discriminative version of the same task?
Interrogative evaluation: For the given generated model output, to what extent can models accurately respond to questions about the content and appropriateness of that output?
The authors find that, in selective evaluation, models meet or exceed humans at generation while lagging at discrimination. In interrogative evaluation, models struggle to answer simple questions about generated content that humans could answer for their own generations.
These observations, to a human, might feel odd. The authors suggest that the (generative) learning objective that defines generative models, which explicitly encourages reconstruction of the training distribution, only implicitly encourages understanding if that furthers the goal. While we might be able to provide context that coaxes models to emulate a specific individual, their behavior tends to look distributionally correct as opposed to individually correct—stylistic and document-wise features take priority over details necessary for understanding tasks.
Why does it matter?
As generative AI systems have become more and more capable in producing outputs that are of human quality, it is tempting to call them “superhuman,” to compare their abilities to those of human artists. As the authors point out, humans’ generative abilities often succeed their discriminative abilities—that is, humans usually need to understand before they can create. While there might be exceptions to this general rule, it is hard for humans to create anything complicated without some level of understanding.
The Generative AI Paradox offers a word of caution against drawing too close a parallel between the capabilities of generative models and human intelligence. Our existing conceptions of intelligence may not apply to today’s models, and we might not want to use these models as guides for our understanding of human intelligence.
Editor Comments
Daniel: This work follows a line of work that has demonstrated how current models’ “understanding” lags and differs from humans’. Recent work has shown that CLIP, while it can correctly match images and labels for those images, lacks compositional understanding: it cannot tell the difference between “man riding horse” and “horse riding man,” for instance. This work re-emphasizes, in an important way, that we should be very careful when applying our notions of “understanding” or “intelligence” to modern AI systems. Generative capabilities are impressive and nothing to scoff at, but we shouldn’t suppose these capabilities imply things about “what’s going on inside” for AI systems the way they do for humans. It’s possible, sure, to develop an understanding of something complicated by seeing examples and spending time staring at it, but it’s likely this is an outcome of understanding (or something conducive to understanding) being your goal in the first place—you’re then likely to attend to things that will help you achieve that goal.
New from the Gradient
Michael Levin & Adam Goldstein: Intelligence and its Many Scales
Jonathan Frankle: From Lottery Tickets to LLMs
Other Things That Caught Our Eyes
News
San Francisco Launches Ground War to Rein in Robotaxis “In the wake of a recent decision by the California Department of Motor Vehicles to block Cruise from operating robotaxis in San Francisco, the city’s lawmakers are set to reignite a ground war against autonomous vehicles with new legislation tightening controls over fleet charging sites.”
ChatGPT Plus members can upload and analyze files in the latest beta “OpenAI is rolling out new beta features for ChatGPT Plus members right now. Subscribers have reported that the update includes the ability to upload files and work with them, as well as multimodal support.”
UK, US, EU and China sign declaration of AI’s ‘catastrophic’ danger “The UK, US, EU and China have all agreed that artificial intelligence poses a potentially catastrophic risk to humanity, in the first international declaration to deal with the fast-emerging technology.”
Aurora, With New Autonomous-Truck Terminal, On Track for Commercial Launch “Aurora Innovation said it’s still on schedule for the commercial launch of its autonomous-truck program at the end of 2024.”
The contentious issue of whether AI art generators violent copyright — since they are by and large trained on human artists’ work, in many cases without their direct affirmative consent, compensation, or even knowledge — has taken a step forward to being settled in the U.S. today.”
Chinese tech giant Alibaba launches upgraded AI model to challenge Microsoft, Amazon “Alibaba on Tuesday launched the latest version of its artificial intelligence model, as the Chinese technology giant looks to compete with U.S. tech rivals such as Amazon and Microsoft. China's biggest cloud computing and e-commerce player announced Tongyi Qianwen 2.”
Justine Bateman's Fight Against Generative AI In Hollywood “The rapid development of generative AI technologies over the last two years, from deepfakes to large language models, has threatened upheavals in many industries.”
Cruise Grew Fast and Angered Regulators. Now It’s Dealing With the Fallout. “Two months ago, Kyle Vogt, the chief executive of Cruise, choked up as he recounted how a driver killed a 4-year-old girl in a stroller at a San Francisco intersection. ‘It barely made the news,’ he said, pausing to collect himself. ‘Sorry. I get emotional.’”
Papers
Daniel: Cameron Jones and Benjamin Bergen released a very interesting preprint detailing the results of an online Turing Test evaluation of GPT-4. Jones and Bergen argue that the test continues to be relevant as a measure of fluent social interaction and deception; they also find that linguistic style and socio-emotional factors were the most cited reasons for GPT-4’s failure to convince a human. This interesting paper argues that AI systems enhance cultural transmission (this reminds me of Ted Underwood’s articulation of generative AI systems as models of culture), and that these models can tell us about imitation and innovation by testing whether they can be used to discover new tools and novel causal structures and contrasting their responses with human childrens’. Finally, this paper refutes Fodor and Pylyshyn’s challenge to neural networks (they argued that ANNs cannot produce novel combinations from known components) by training neural networks to develop compositional skills.
Closing Thoughts
Have something to say about this edition’s topics? Shoot us an email at editor@thegradient.pub and we will consider sharing the most interesting thoughts from readers to share in the next newsletter! For feedback, you can also reach Daniel directly at dbashir@hmc.edu or on Twitter. If you enjoyed this newsletter, consider donating to The Gradient via a Substack subscription, which helps keep this grad-student / volunteer-run project afloat. Thanks for reading the latest Update from the Gradient!
Missing a bit on the OpenAI dev day maybe ;)