Update #38: Lethal Robots in SF and CICERO (w/ co-author Hugh Zhang)
In which we discuss a pending decision in SF on the deployment of robots with lethal capabilities and Meta's AI system that beats humans in Diplomacy, with Gradient editor + co-author Hugh Zhang
Welcome to the 38th update from the Gradient! If you were referred by a friend, subscribe and follow us on Twitter!
Want to write with us? Send a pitch using this form :)
News Highlight: San Francisco Considers Allowing Police To Deploy Robots With Lethal Capabilities
The decision is not final and requires another vote and the Mayor’s approval before it can be enacted
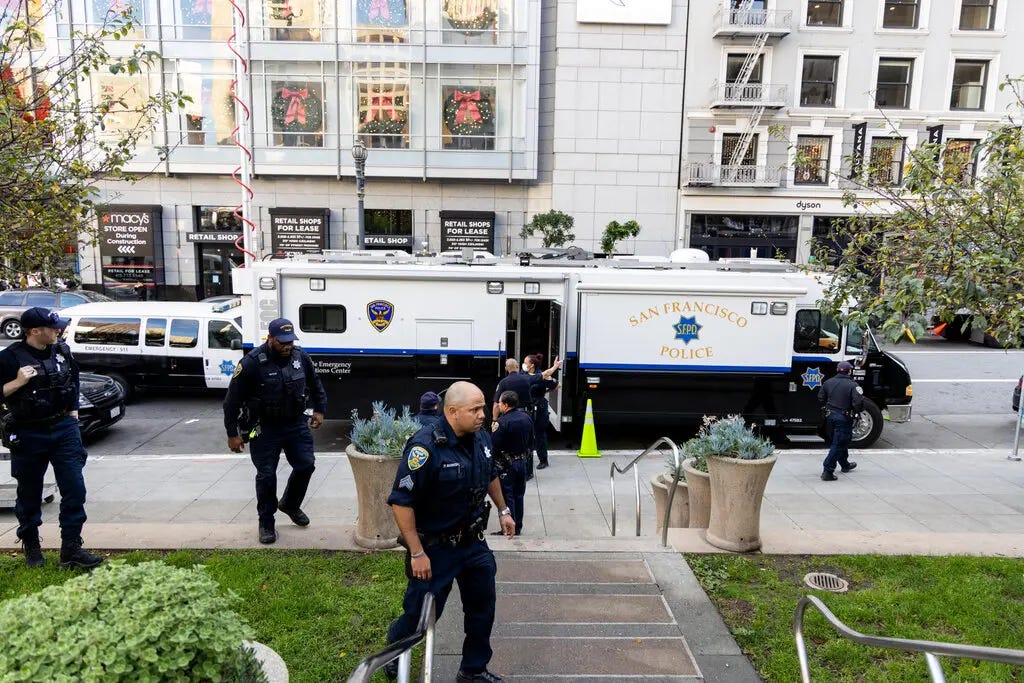
Summary
The San Francisco Board of Supervisors passed a policy proposal potentially allowing the police to use lethal robots in “emergency situations”. The proposal states that these robots could be used in situations involving a terrorist attack or an active shooter, putting police officers out of harm’s way. The highly controversial policy is the first of its kind to be considered in a US city and if implemented, could pave the way for more cities to follow suit.
Background
The militarization of police in the United States has been a deeply debated issue that intensifies the already tense relationship between the police and civilians in America. In an attempt to address this issue, the state of California passed a law last year requiring all law enforcement agencies to seek approval from their civic bodies before using military-style equipment. Following this, the San Francisco Police Department submitted a policy seeking permission to use an array of equipment in its possession including a sound cannon, a BearCat armored vehicle, a launcher to deploy chemical agents, and – 17 robots. According to the SFPD, some of these robots can climb stairs, others with tank treads can defuse bombs, or deliver an instantaneous video and audio feed.
The department clarified that the robots are currently not weaponized, and there are no plans at the moment to weaponize them either. That being said, the policy seeks approval to potentially weaponize these robots and use them in situations when “risk of loss of life to members of the public or officers is imminent and officers cannot subdue the threat after using alternative force options or de-escalation tactics options or conclude that they will not be able to subdue the threat after evaluating alternative force options and de-escalation tactics” (NYT, Online).
This idea of weaponizing robots is not entirely new – the Dallas Police Department first used a robot to take down a gunman who was suspected of killing five police officers (CNN, 2016). In 2021, the New York Police Department returned its “robot dog” (Spot, from Boston Dynamics) after receiving severe backlash from the community. While these robots were not weaponized, they were used in hostage situations and public buildings, but were eventually terminated due to concerns ranging from cost, creepiness and endangerment of civil liberties (Scientific American, 2021). We are yet to see how the vote turns out on Dec 6th for the city of San Francisco, potentially altering the landscape of policing in the age of AI.
Why does it matter?
Weaponizing robots brings many ethical challenges, especially when teleoperated by law enforcement. Many are concerned that it will decrease accountability and make applying lethal force easier for law enforcement. Paul Scharre, the vice president and director of studies at the Center for a New American Security and the author of “Army of None: Autonomous Weapons and the Future of War”, discussed this problem in a comment to the New York Times, noting that since robots can create distance between law enforcement agents and threats, they now should have the opportunity to use non-lethal force such as flash bangs to incapacitate someone instead of using lethal force. Proponents of the policy view it as an appropriate use of modern day technologies with the goal of improving public safety. Perhaps some different questions need to be asked to analyze this policy, such as, are there any instances in which the police – not the military – had to use advanced technologies such as sound cannons, armored vehicles or teleoperated robots? If so, what is it about America that led to these situations being a part of our reality? And are military grade weapons the answer to solving that root cause? The upcoming vote has the potential to set a strong precedent about the use of AI by law enforcement in the United States and initiate new discussions in the space of ethics in robotics.
Editor Comments
Hugh: I strongly disagree with the proposal. While it is understandable that law enforcement may want to protect officers and the public in dangerous situations, using robots to apply lethal force is not the solution. For instance, why not simply use non-lethal weapons, especially as using a robot prevents human lives from being in the line of fire?
Research Highlight: Cicero: Human-level play in Diplomacy
Summary
The Meta Fundamental AI Research Diplomacy Team has developed Cicero — the first AI model that achieves human level performance in the strategy game Diplomacy. This model both cooperates and competes against human players through communicating in natural language and strategizing to make actions in games.
Overview
The team tested Cicero in 40 games on webDiplomacy.net, and found that Cicero achieves human level performance. Indeed, of all participants with at least two games played, Cicero ranked in the top 10%. Further, Cicero won an 8-game tournament of 21 participants, and achieved an average score across 40 games that was more than two times the average score of its opponents. Identities were anonymous during these games, and only one player out of 82 mentioned suspicion (in a post-game chat) that Cicero may be a bot. From the chat logs, we can see that Cicero coordinates and negotiates with human players in Diplomacy-specific jargon, and is capable of working towards achieving goals with this strategic communication.
Cicero combines a natural language dialogue model with strategic reasoning modules. It generates messages with a pre-trained Transformed-based model that was further finetuned on messages from human-only Diplomacy games. The dialogue generation is conditioned on the message history, game state, and predicted intents of other players. Generated messages are chosen with the help of several filters, which are designed to eliminate incomprehensible, inconsistent, toxic, or strategically poor messages. Cicero also has a strategic reasoning module, trained with self-play reinforcement learning, which predicts policies of other players and selects actions for itself to execute.
Why does it matter?
Related AI methods have achieved expert performance in several types of games, such as Chess, Go, and StarCraft. However, these are mostly two player zero sum games, which do not require coordination with other actors to solve, and hence are often easier to solve through reinforcement learning and self-play. On the other hand, proper communication with humans is essential to success in Diplomacy; thus, Cicero learns to cooperate, negotiate, and coordinate with humans through natural language in order to achieve its goals.
Author Q&A
For more thoughts on the work, we spoke to the EXTREMELY COOL Hugh Zhang, an editor at The Gradient who was part of the Cicero team. All thoughts are his alone, and do not necessarily represent or agree with the opinions of Meta or other members of the team.
Q: Did you expect AI success in Diplomacy within these timelines? How did others feel about this?
A: There was a range of opinions on the team. Some people were more skeptical than others, but mostly everyone eventually came to the same conclusion, at least by the time the paper was published :D. I consider myself a fairly optimistic believer in scaling laws, so I think these timelines were more or less what I expected when Noam told me about the project in late 2019. Even taking the outside view, seeing things like GPT-3 and superhuman AI agents in basically every (non language based) game makes it fairly natural to believe that a combination of these two research paths (i.e. full press Diplomacy) cannot be too far away.
Q: What AI advances were key to Cicero’s success?
A: Both game theory and language models were clearly very important, but if I had to pick, I’d say developments in language modeling were more critical. A bot that could use language models circa ~2022 and game theory circa ~2017 would probably be a passable, but not great Diplomacy player. The reverse likely wouldn’t be able to get off the ground.
Q: Hugh think fast what’s a cool paper u have seen recently
A: https://www.cs.toronto.edu/~hinton/FFA13.pdf
Reddit AMA:

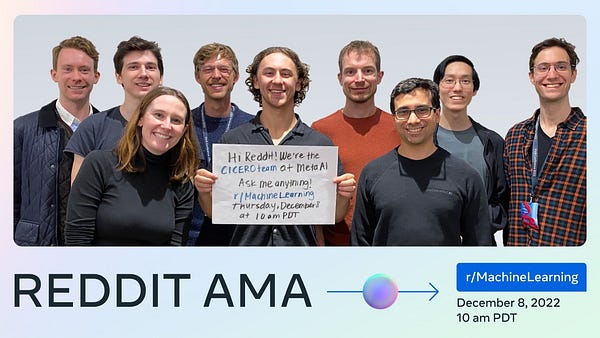
New from the Gradient
François Chollet: Keras and Measures of Intelligence
Other Things That Caught Our Eyes
News
AI Is Terrible at Detecting Misinformation. It Doesn’t Have to Be. “Elon Musk has said he wants to make Twitter “the most accurate source of information in the world.” I am not convinced that he means it, but whether he does or not, he’s going to have to work on the problem; a lot of advertisers have already made that pretty clear.”
Now AI can write students’ essays for them, will everyone become a cheat? “Parents and teachers across the world are rejoicing as students have returned to classrooms. But unbeknownst to them, an unexpected insidious academic threat is on the scene: a revolution in artificial intelligence has created powerful new automatic writing tools.”
How the World Cup’s AI instant replay works “A new hyper-accurate technology, and referees' eternal quest for objectivity.“
Amazon’s Quest for the ‘Holy Grail’ of Robotics “Automating some warehouse functions could let the giant do more with its existing workforce—or the retailer may not require all the humans it employs today“
Papers
RGB no more: Minimally-decoded JPEG Vision Transformers Most neural networks for computer vision are designed to infer using RGB images. However, these RGB images are commonly encoded in JPEG before saving to disk; decoding them imposes an unavoidable overhead for RGB networks. Instead, our work focuses on training Vision Transformers (ViT) directly from the encoded features of JPEG… Due to how these encoded features are structured, CNNs require heavy modification to their architecture to accept such data. Here, we show that this is not the case for ViTs. In addition, we tackle data augmentation directly on these encoded features, which to our knowledge, has not been explored in-depth for training in this setting. With these two improvements -- ViT and data augmentation -- we show that our ViT-Ti model achieves up to 39.2% faster training and 17.9% faster inference with no accuracy loss compared to the RGB counterpart.
One is All: Bridging the Gap Between Neural Radiance Fields Architectures with Progressive Volume Distillation In this paper, we propose Progressive Volume Distillation (PVD), a systematic distillation method that allows any-to-any conversions between different architectures, including MLP, sparse or low-rank tensors, hashtables and their compositions. PVD consequently empowers downstream applications to optimally adapt the neural representations for the task at hand in a post hoc fashion. The conversions are fast, as distillation is progressively performed on different levels of volume representations, from shallower to deeper. We also employ special treatment of density to deal with its specific numerical instability problem. Empirical evidence is presented to validate our method on the NeRF-Synthetic, LLFF and TanksAndTemples datasets. For example, with PVD, an MLP-based NeRF model can be distilled from a hashtable-based Instant-NGP model at a 10X~20X faster speed than being trained the original NeRF from scratch, while achieving a superior level of synthesis quality.
Tweets
Closing Thoughts
Have something to say about this edition’s topics? Shoot us an email at editor@thegradient.pub and we will consider sharing the most interesting thoughts from readers to share in the next newsletter! For feedback, you can also reach Daniel directly at dbashir@hmc.edu or on Twitter. If you enjoyed this newsletter, consider donating to The Gradient via a Substack subscription, which helps keep this grad-student / volunteer-run project afloat. Thanks for reading the latest Update from the Gradient!