Update #67: AI Confuses Elections and Conversational Diagnostic AI
AI-generated content causes and portends confusion in national elections, and Google researchers develop an LLM-based AI system for diagnosing patients.
Welcome to the 67th update from the Gradient! If you’re new and like what you see, subscribe and follow us on Twitter :) You’ll need to view this post on Substack to see the full newsletter!
We’re recruiting editors! If you’re interested in helping us edit essays for our magazine, reach out to editor@thegradient.pub.
Want to write with us? Send a pitch using this form.
News Highlight: AI-generated content is driving confusion and deception in elections around the world
Summary
In 2024, more than half the world's population will be eligible to participate in national elections, including those in the United States, United Kingdom and India. As these elections unfold, citizens are left to wrestle with a rise in AI-generated content driving mass confusion, misleading voters, and functioning as a plausibly deniable scapegoat for real damaging content and allegations. Some examples of those incidents and allegations from the past year include:
Robocalls from President Joe Biden encouraging voters to stay home days before primary elections
Politicians from Indian state of Tamil Nadu claiming leaked recordings alleging illegal amassing of $3.6 Billion as “machine generated”
Donald Trump claiming an ad which used widely reported clips of public gaffes is “fake television” made from AI
A Taiwanese politician dismissing video evidence of an extramarital affair as AI generated
Experts who spoke to the Washington Post about these incidents are claiming AI is muddying the waters of perceived reality and creating a “liar’s dividend”. In her interview with the Washington Post, UC Berkeley professor Hany Farid describes the liar’s dividend: when politicians are caught doing something awful, they have a plausibly deniable claim under the guise of AI-generated deep fakes. While there has been little evidence of deep fake propaganda prior to this year, the Biden voice clone may signal changing times. As elections season progresses, voters, the media, and relevant law enforcement agencies should remain vigilant about the rise in artificially generated content and attempts to capitalize on the chaos of generated content to further distort reality.
Overview
On January 21st, two days before the New Hampshire’s Presidential Primary election, voters received a robocall from an AI-generated voice clone of President Biden, encouraging them to skip participating in the primary. They were told that their vote matters in November, not on Tuesday, (the date of the primary). These calls prompted the New Hampshire Department of Justice to kick off an ongoing investigation into an “unlawful attempt to disrupt the New Hampshire Democratic Presidential Primary Election and to suppress New Hampshire voters.” The robocall is not the only instance of AI-generated content influencing the New Hampshire Primary. At around the same time, OpenAI banned the development account of a Super PAC that supports Democratic Presidential Candidate Dean Phillips. The account was banned for creating an AI chatbot of Phillips, in violation of OpenAI’s ban of their technology in political campaigns.
As the quality of machine learning models improves, so too does the likelihood that voters would struggle to disentangle AI generated content from reality. Recent studies have shown humans struggle with identifying AI generated images, writings, and faces with accuracies ranging from 40-60% depending on the task at hand. To understand the practical impact from our inability to identify artificial content we can consider some examples regarding Donald Trump. It should be noted that this phenomenon is not unique to him nor American politics.
Recently the actor Mark Ruffallo apologized for sharing sensationalized AI generated photos of Donald Trump with young women at Jeffery Epstein’s “Fantasy Island”. While these images could be seemingly plausible given Trump and Epstein’s decades long friendship, they turned out to be AI-generated. Examples such as this undermine the trust of Ruffallo and provide an air of credibility to claims that Donald Trump is victim to a host of fake AI generated content. This recently manifested when Donald Trump claimed that a new ad featuring embarrassing public gaffes of his was AI generated. In actuality, the gaffes were from widely reported clips, many of which predate the authoring of some of the most foundational papers in AI.
Why does it matter?
On one hand, we have legitimately fake generated content used to suppress voters and violate the civil rights of hundreds of thousands in New Hampshire. On the other hand, we have the pretext of AI-generated content being used to dismiss legitimate primary source reportings as fake undermining voters' trust in media and political entities. Together, we could be seeing the stage set for a mass deluge of AI-generated content designed to influence elections and confuse voters around the world. We should see the recent fake robot calls in New Hampshire and dismissals of widely documented events as AI generated as a clarion call for the times ahead.
Now more than ever, media organizations, influencers, celebrities, and voters need to engage critically with the media content that they see and share, considering both the content and context of the media. On top of increased critical analysis and scrutiny, we should be applauding the decisions of companies like OpenAI for banning the use of their technologies in political campaigns and upping criticisms of those that don’t care. Finally, voters should be turning to their own governments for stricter regulations and enforcement mechanisms designed to stem the numerous harms from generative AI. Some of those risks include directing voters to stay home, misinforming voters about when or how to vote, or via a liar’s dividend where those in power use the speculation of generative AI to undermine primary source reporting and attempt to distort shared understandings of reality. Absent any changes to the regulatory landscape (particularly in the US and EU), voters should prepare to be more vigilant than ever when engaging with political content.
Research Highlight: Towards Conversational Diagnostic AI
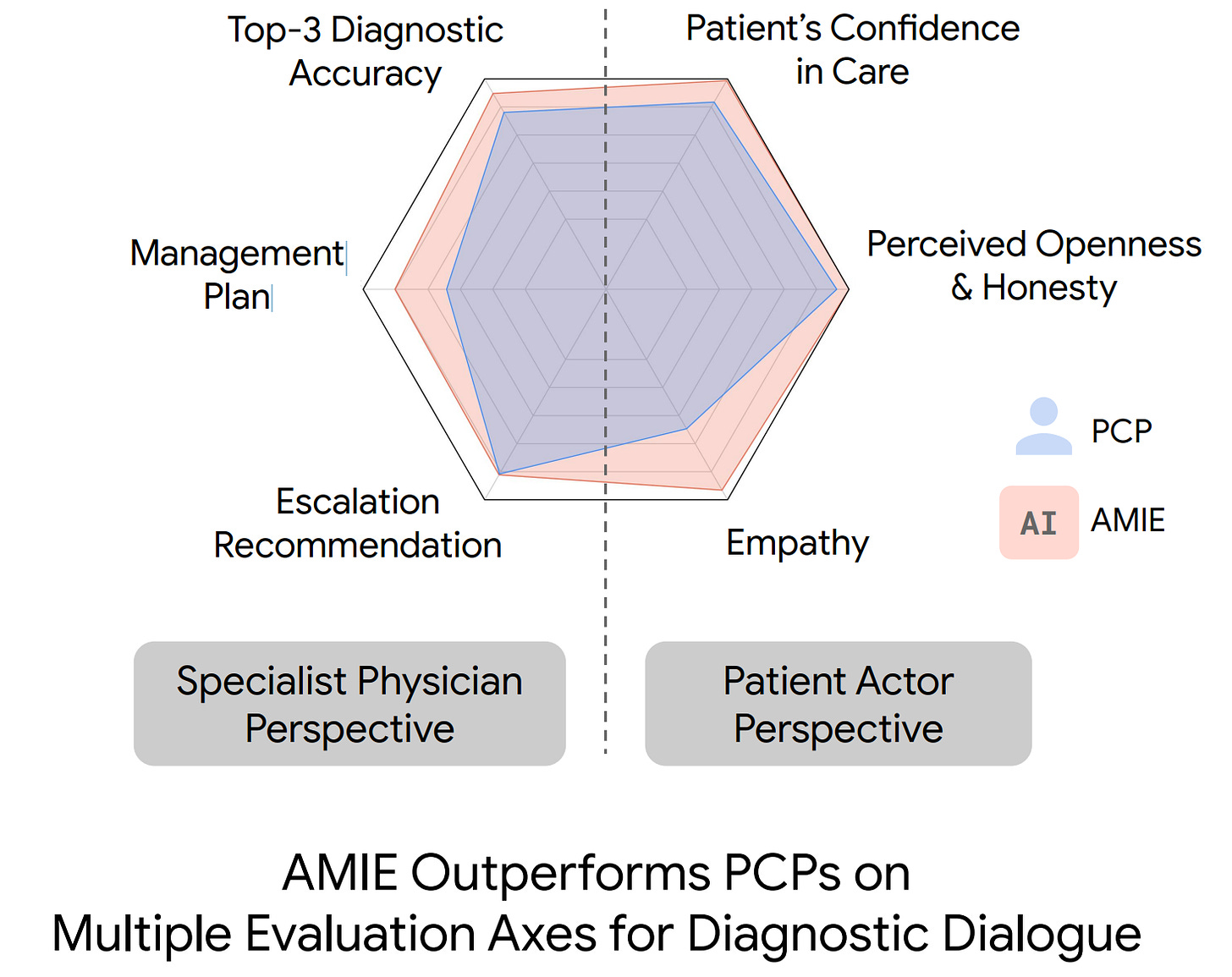
Summary
In medicine, the vital interaction between physicians and patients shapes the foundation of healthcare. Recently, a team from Google Research and Google DeepMind introduced an Articulate Medical Intelligence Explorer (AMIE), an AI system trained to have conversations to diagnose patients by learning from real doctor-patient dialogues and simulations. The results are striking—AMIE outperforms primary care physicians (PCPs) with greater diagnostic accuracy across various medical axes, including empathy, management plan, openness and honesty, and more.
As healthcare continues to evolve, the findings from this research mark a milestone in the pursuit of developing AI systems that can truly complement the indispensable role of doctors in delivering high-quality, patient-centric care.
Overview
The essential dialogue between physicians and patients forms the bedrock of effective and compassionate healthcare. Acknowledged as the physician's most potent instrument, the medical interview plays a pivotal role, contributing significantly to diagnoses—60-80% in certain contexts. Despite clinicians' proficiency in this diagnostic dialogue, global access to such expertise remains sporadic. Recognizing the global scarcity of access to clinicians' expertise, the authors of ‘Towards Conversational Diagnostic AI‘ introduce AMIE (Articulate Medical Intelligence Explorer), the first large language model (LLM) based AI system for clinical history-taking and diagnostic reasoning. AMIE capitalizes on the recent advancements in general-purpose LLMs, showcasing advanced abilities for engaging in naturalistic conversations.
AMIE's development involves training with a diverse suite of real-world datasets, including medical question-answering (multiple choice), reasoning (long-form answers), and transcribed medical conversations. The dialogue dataset spans across 51 specialties and 168 conditions, covering various in-person clinical visits, with an average of 149.8 turns per conversation. Metadata includes patient demographics, visit reasons, and diagnosis types, serving as key essential features for personalization.
However, passively modeling this data presents two key challenges 1) inherent noise in the data like slang, sarcasm etc., and 2) the data do not capture a vast range of medical conditions and scenarios, especially rare diseases. To overcome these challenges, a self-play based simulated learning environment was designed as shown in the Figure above.
This virtual care setting allowed iterative fine-tuning of AMIE by combining simulated dialogues with the static corpus of medical QA, reasoning, summarization, and real-world dialogue data. The self-play process involved both an "inner" loop for refining behavior on simulated conversations and an "outer" loop incorporating refined dialogues into subsequent fine-tuning iterations, creating a continuous learning cycle. In order to generate less noisy dialogues at scale, this pipeline includes three key components:
Vignette Generator: Generates realistic patient scenarios containing patient history, patient metadata, patient questions as well as an associated diagnosis and plan;
Simulated Dialogue Generator: Using the out of Vignette Generator, this LLM agent simulates a realistic dialogue between a patient and a doctor by rendering three more LLM agents - doctor, patient and a moderator agent
Self-play Critic: This LLM agent serves as a critic to the dialogues generated by the doctor agent in the above step, providing feedback for self improvement.
AMIE is compared to real clinicians through a randomized crossover study, simulating a remote Objective Structured Clinical Examination (OSCE). This study involves over 20 board-certified primary care physicians (PCPs) and 20 validated patient actors across India and Canada.
The results were impressive. As shown in the figure below (a), AMIE demonstrates significantly higher diagnostic accuracy than PCPs. To investigate the reason for this gain, the authors compare AMIE’s diagnoses based on its own consultations with those generated from the PCP consultations. As shown in figure (b), the diagnostic performance remains almost the same whether it processes information from its own dialogue or from the PCP's conversation. This shows that the actual gain in performance is due to AMIE being better than PCPs at producing an accurate/complete differential diagnosis.
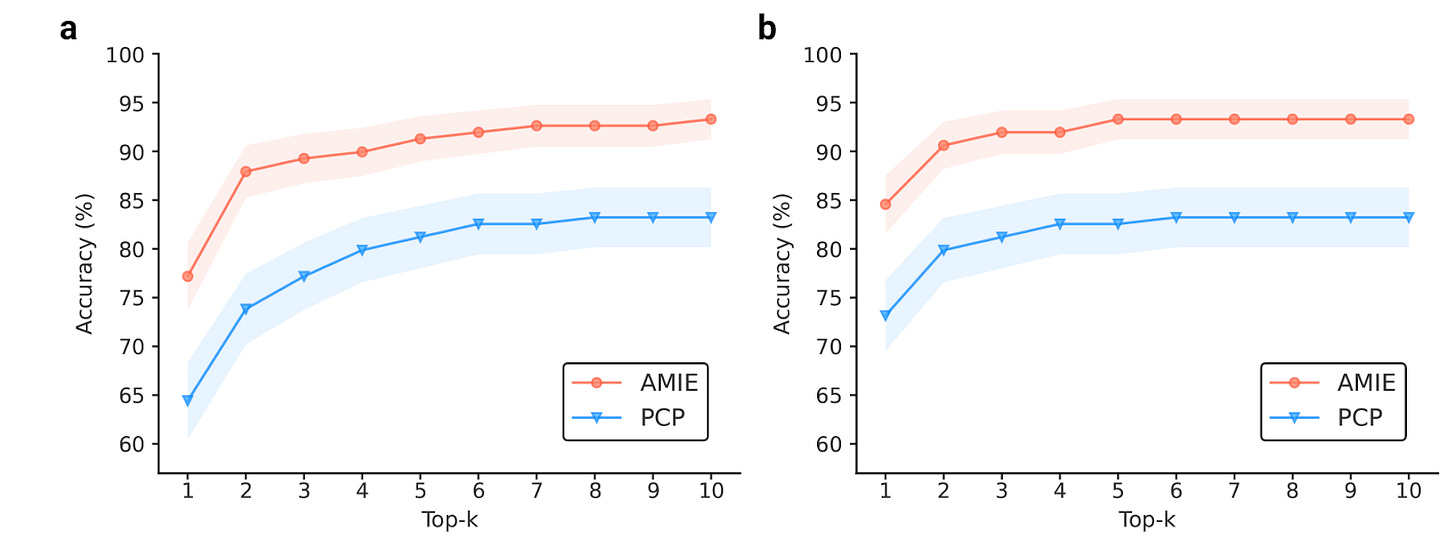
Further, as shown in the figure below, specialists consistently rated AMIE's responses higher than those from PCPs on 28 out of 32 evaluation criteria. These axes include consultation, empathy, diagnosis and management plan.
However, one should be cautious while interpreting these results. For instance, clinicians in this simulated setting are constrained to synchronous text-chat, a method that facilitates extensive LLM-patient interactions on a large scale but deviates from the typical dynamics of clinical practice. Further, the patient actors may have articulated their symptoms with an unusually rich level of detail or structure, which wouldn’t be ordinarily expected.
Why does it matter?
The ability of medical AI systems to engage in conversational interactions with a nuanced understanding of clinical history and diagnostics holds immense significance for healthcare. This research showcases the promising capabilities of Large Language Models (LLMs) in areas of clinical history-taking and diagnostic dialogue. By leveraging vast medical knowledge and exhibiting empathy and trust in conversations, these systems have the potential to be integral components of the next generation of learning health systems. However, it's crucial to approach this potential with caution, recognizing that transitioning from experimental simulations to practical, real-world tools demands thorough research and development. Thus, ensuring the reliability and safety of such technology is paramount for its successful integration into healthcare practices.
New from the Gradient
Benjamin Breen: The Intersecting Histories of Psychedelics and AI Research
Ted Gibson: The Structure and Purpose of Language
Other Things That Caught Our Eyes
News
Introducing Stable LM 2 1.6B “Stable LM 2 1.6B is a state-of-the-art 1.6 billion parameter small language model trained on multilingual data in English, Spanish, German, Italian, French, Portuguese, and Dutch.”
Voice cloning startup ElevenLabs lands $80M, achieves unicorn status “ElevenLabs, a startup developing AI-powered tools to create and edit synthetic voices, today announced that it closed an $80 million Series B round co-led by prominent investors, including Andreessen Horowitz, former GitHub CEO Nat Friedman and entrepreneur Daniel Gross.”
A ‘Shocking’ Amount of the Web Is Already AI-Translated Trash, Scientists Determine “A ‘shocking’ amount of the internet is machine-translated garbage, particularly in languages spoken in Africa and the Global South, a new study has found.”
San Francisco takes legal action over ‘unsafe,’ ‘disruptive’ self-driving cars “San Francisco is suing the state over an August decision to allow two major autonomous car companies to expand in the city.”
Nvidia, Microsoft, Google, and others partner with US government on AI research program “The National Science Foundation (NSF) announced on Wednesday that it is teaming up with some of the biggest names in tech to launch the National Artificial Intelligence Research Resource (NAIRR) pilot program.”
Man sues Macy’s, saying false facial recognition match led to jail assault “A man was sexually assaulted in jail after being falsely accused of armed robbery due to a faulty facial recognition match, his attorneys said, in a case that further highlights the dangers of the technology’s expanding use by law enforcement.”
New Texas Center Will Create Generative AI Computing Cluster Among Largest of Its Kind “The University of Texas at Austin is creating one of the most powerful artificial intelligence hubs in the academic world to lead in research and offer world-class AI infrastructure to a wide range of partners.”
Most Top News Sites Block AI Bots. Right-Wing Media Welcomes Them “As media companies haggle licensing deals with artificial intelligence powerhouses like OpenAI that are hungry for training data, they’re also throwing up a digital blockade.”
Akutagawa Prize draws controversy after win for work that used ChatGPT “In recent years, the Akutagawa Prize has diversified its recipient pool: In 2022, the shortlist was all female writers, last year Saou Ichikawa was the first author with a severe physical disability to win and this week marked the first time artificial intelligence walked away with a piece of the prestigious literary award.”
Papers
Daniel: MambaByte is a really interesting paper that explores token-free language models, with the goal of removing bias from subword tokenization. This study on using transformer-based language models to emulate recursive computation provides a powerful conceptual framework for thinking about structural recursion in models. Finally, about a month ago, Deb Raji and Roel Dobbe uploaded their (older) paper Concrete Problems in AI Safety, Revisited, which argues for an expanded socio-technical framing to understand how AI systems and implemented safety mechanisms fail and succeed in real life.
Closing Thoughts
Have something to say about this edition’s topics? Shoot us an email at editor@thegradient.pub and we will consider sharing the most interesting thoughts from readers to share in the next newsletter! For feedback, you can also reach Daniel directly at dbashir@hmc.edu or on Twitter. If you enjoyed this newsletter, consider donating to The Gradient via a Substack subscription, which helps keep this grad-student / volunteer-run project afloat. Thanks for reading the latest Update from the Gradient!